Discover more about PhenoRob: The PhenoRob paper videos present the most important findings published in high-ranked journals.

Effects of spring wheat / faba bean mixtures on early crop development
Madhuri Paul is a PhD student at the Institute Agroecology and Organic Farming, University of Bonn. M. R. Paul, D. T. Demie, S. J. Seidel, and T. F. Döring, “Effects of spring wheat / faba bean mixtures on early crop development,” Plant and Soil, 2023. [doi:10.1007/s11104-023-06111-6]
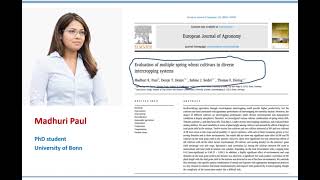
Evaluation of multiple spring wheat cultivars in diverse intercropping systems
Madhuri Paul is a PhD student at the Institute Agroecology and Organic Farming, University of Bonn. M. R. Paul, D. T. Demie, S. J. Seidel, and T. F. Döring, “Evaluation of multiple spring wheat cultivars in diverse intercropping systems,” European Journal of Agronomy, vol. 152, p. 127024, 2024. [doi:https://doi.org/10.1016/j.eja.2023.127024]
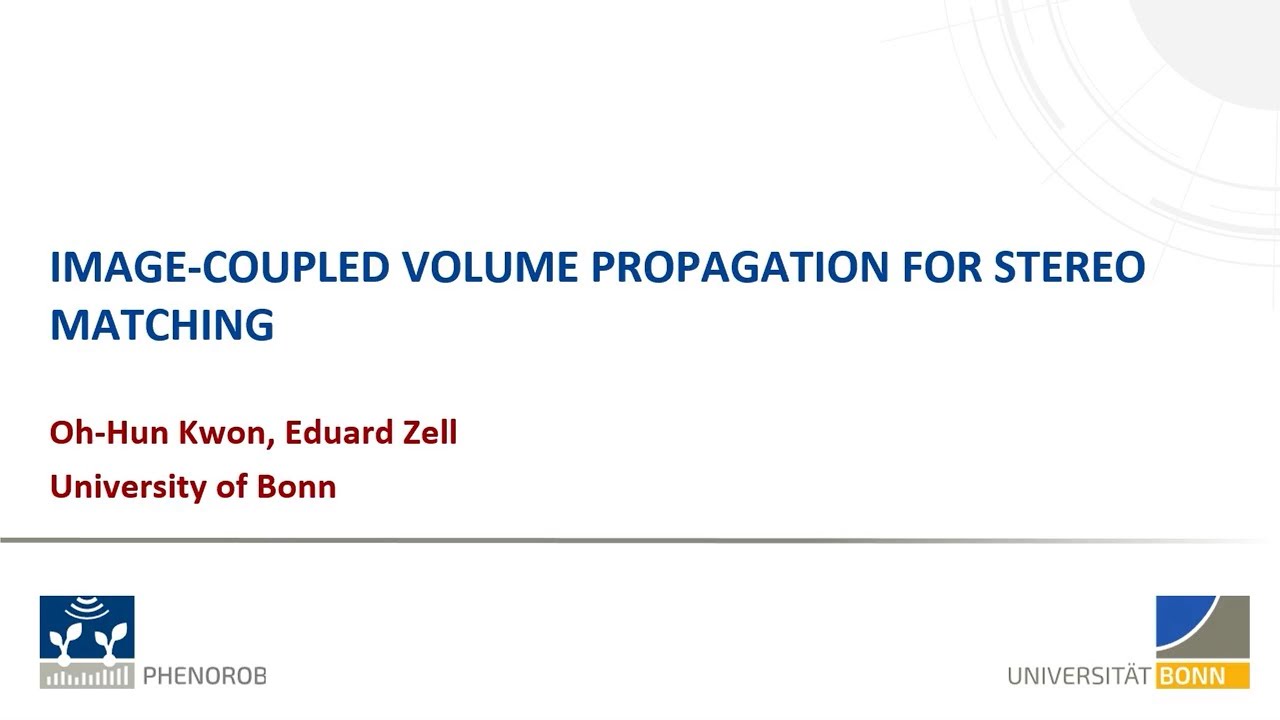
Image-Coupled Volume Propagation for Stereo Matching by Oh-Hun Kwon and Eduard Zell
This trailer video is based on following publication: O. Kwon and E. Zell, “Image-Coupled Volume Propagation for Stereo Matching,” in 2023 IEEE International Conference on Image Processing (ICIP), 2023, pp. 2510-2514. doi:10.1109/ICIP49359.2023.10222247

DawnIK: Decentralized Collision-Aware Inverse Kinematics Solver for Heterogeneous Multi-Arm Systems
This video demonstrates the work presented in our paper “DawnIK: Decentralized Collision-Aware Inverse Kinematics Solver for Heterogeneous Multi-Arm Systems” by S. Marangoz, R. Menon, N. Dengler, and M. Bennewitz, submitted to IEEE-RAS International Conference on Humanoid Robots (Humanoids), 2023. With collaborative service robots gaining traction, different robotic systems have to work in close proximity. This means that the current inverse kinematics approaches do not have only to avoid collisions with themselves but also collisions with other robot arms. Therefore, we present a novel approach to compute inverse kinematics for serial manipulators that take into account different constraints while trying to reach a desired end-effector pose that avoids collisions with themselves and other arms. We formulate different constraints as weighted cost functions to be optimized by a non-linear optimization solver. Our approach is superior to a state-of-the-art inverse kinematics solver in terms of collision avoidance in the presence of multiple arms in confined spaces with no collisions occurring in all the experimental scenarios. When the probability of collision is low, our approach shows better performance at trajectory tracking as well. Additionally, our approach is capable of simultaneous yet decentralized control of multiple arms for trajectory tracking in intersecting workspace without any collisions. Paper link https://arxiv.org/abs/2307.12750

PermutoSDF: Fast Multi-View Reconstruction with Implicit Surfaces using Permutohedral Lattices
Neural radiance-density field methods have become increasingly popular for the task of novel-view rendering. Their recent extension to hash-based positional encoding ensures fast training and inference with visually pleasing results. However, density-based methods struggle with recovering accurate surface geometry. Hybrid methods alleviate this issue by optimizing the density based on an underlying SDF. However, current SDF methods are overly smooth and miss fine geometric details. In this work, we combine the strengths of these two lines of work in a novel hash-based implicit surface representation. We propose improvements to the two areas by replacing the voxel hash encoding with a permutohedral lattice which optimizes faster, especially for higher dimensions. We additionally propose a regularization scheme which is crucial for recovering high-frequency geometric detail. We evaluate our method on multiple datasets and show that we can recover geometric detail at the level of pores and wrinkles while using only RGB images for supervision. Furthermore, using sphere tracing we can render novel views at 30 fps on an RTX 3090. The paper, animations, and code is available at https://radualexandru.github.io/permuto_sdf
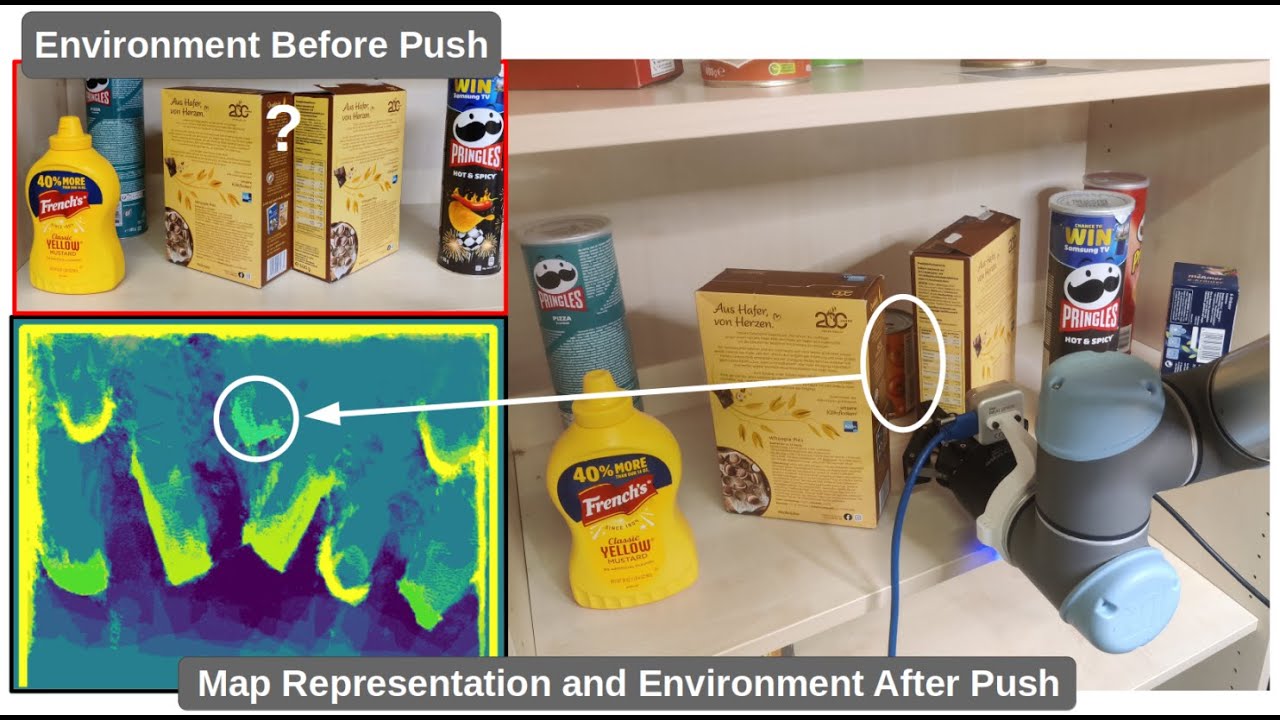
Viewpoint Push Planning for Mapping of Unknown Confined Spaces
This video demonstrates the work presented in our paper “Viewpoint Push Planning for Mapping of Unknown Confined Spaces” by N. Dengler, S. Pan, V. Kalagaturu, R. Menon, M. Dawood and Maren Bennewitz, submitted to the International Conference on Intelligent Robots and Systems (IROS), 2023. Paper link: https://arxiv.org/pdf/2303.03126.pdf The mapping of confined spaces such as shelves is an especially challenging task in the domain of viewpoint planning, since objects occlude each other and the scene can only be observed from the front, thus with limited possible viewpoints. In this video, we show our deep reinforcement learning framework that generates promising views aiming at reducing the map entropy. Additionally, the pipeline extends standard viewpoint planning by predicting adequate minimally invasive push actions to uncover occluded objects and increase the visible space. Using a 2.5D occupancy height map as state representation that can be efficiently updated, our system decides whether to plan a new viewpoint or perform a push. As the real-world experimental results with a robotic arm show, our system is able to significantly increase the mapped space compared to different baselines, while the executed push actions highly benefit the viewpoint planner with only minor changes to the object configuration. Code: https://github.com/NilsDengler/view-point-pushing

NBV-SC: Next Best View Planning based on Shape Completion for Fruit Mapping and Reconstruction
This video demonstrates the work presented in our paper “NBV-SC: Next Best View Planning based on Shape Completion for Fruit Mapping and Reconstruction” by R. Menon, T. Zaenker, N. Dengler and M. Bennewitz, submitted to the International Conference on Intelligent Robots and Systems (IROS), 2023. State-of-the-art viewpoint planning approaches utilize computationally expensive ray casting operations to find the next best viewpoint. In our paper, we present a novel viewpoint planning approach that explicitly uses information about the predicted fruit shapes to compute targeted viewpoints that observe as yet unobserved parts of the fruits. Furthermore, we formulate the concept of viewpoint dissimilarity to reduce the sampling space for more efficient selection of useful, dissimilar viewpoints. In comparative experiments with a state-of-the-art viewpoint planner, we demonstrate improvement not only in the estimation of the fruit sizes, but also in their reconstruction, while significantly reducing the planning time. Finally, we show the viability of our approach for mapping sweet peppers plants with a real robotic system in a commercial glasshouse. Paper link https://arxiv.org/abs/2209.15376
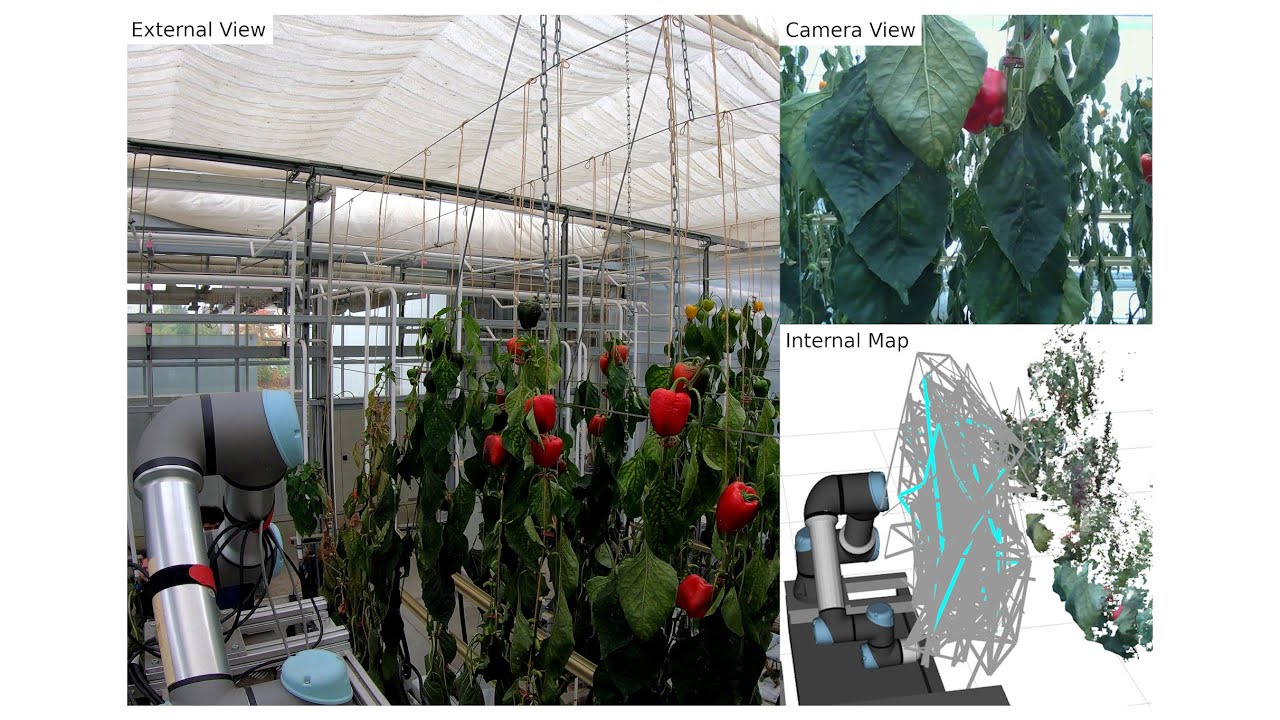
Graph-based View Motion Planning for Fruit Detection
This video demonstrates the work presented in our paper “Graph-based View Motion Planning for Fruit Detection” by T. Zaenker, J. Rückin, R. Menon, M. Popović, and M. Bennewitz, submitted to the International Conference on Intelligent Robots and Systems (IROS), 2023. Paper link: https://arxiv.org/abs/2303.03048 The view motion planner generates view pose candidates from targets to find new and cover partially detected fruits and connects them to create a graph of efficiently reachable and information-rich poses. That graph is searched to obtain the path with the highest estimated information gain and updated with the collected observations to adaptively target new fruit clusters. Therefore, it can explore segments in a structured way to optimize fruit coverage with a limited time budget. The video shows the planner applied in a commercial glasshouse environment and in a simulation designed to mimic our real-world setup, which we used to evaluate the performance. Code: https://github.com/Eruvae/view_motion_planner
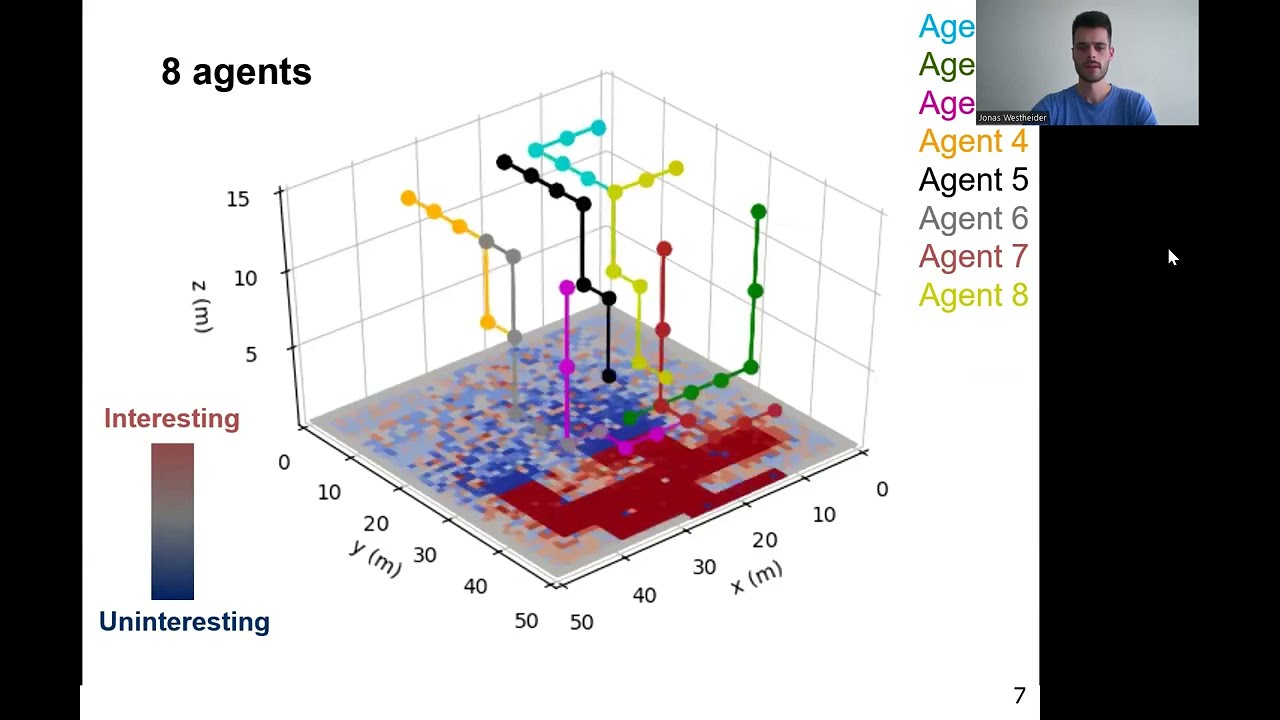
Multi-UAV Adaptive Path Planning Using Deep Reinforcement Learning
Jonas Westheider is a PhD Student at the Institute of Geodesy and Geoinformation (IGG), University of Bonn. Westheider, J., Rückin, J., & Popović, M., “Multi-UAV Adaptive Path Planning Using Deep Reinforcement Learning”. arXiv preprint arXiv:2303.01150. doi: https://doi.org/10.1016/j.robot.2022.104288

Adoption and diffusion of digital farming technologies
Dr. Sebastian Rasch is a Postdoctoral Researcher at the Institute for Food and Resource Economics (ILR), University of Bonn. Shang, L., Heckelei, T., Gerullis, M. K., Börner, J., & Rasch, S. 2021. Adoption and diffusion of digital farming technologies – integrating farm-level evidence and system interaction. Agricultural Systems, 190, 103074. doi: https://doi.org/10.1016/j.agsy.2021.103074
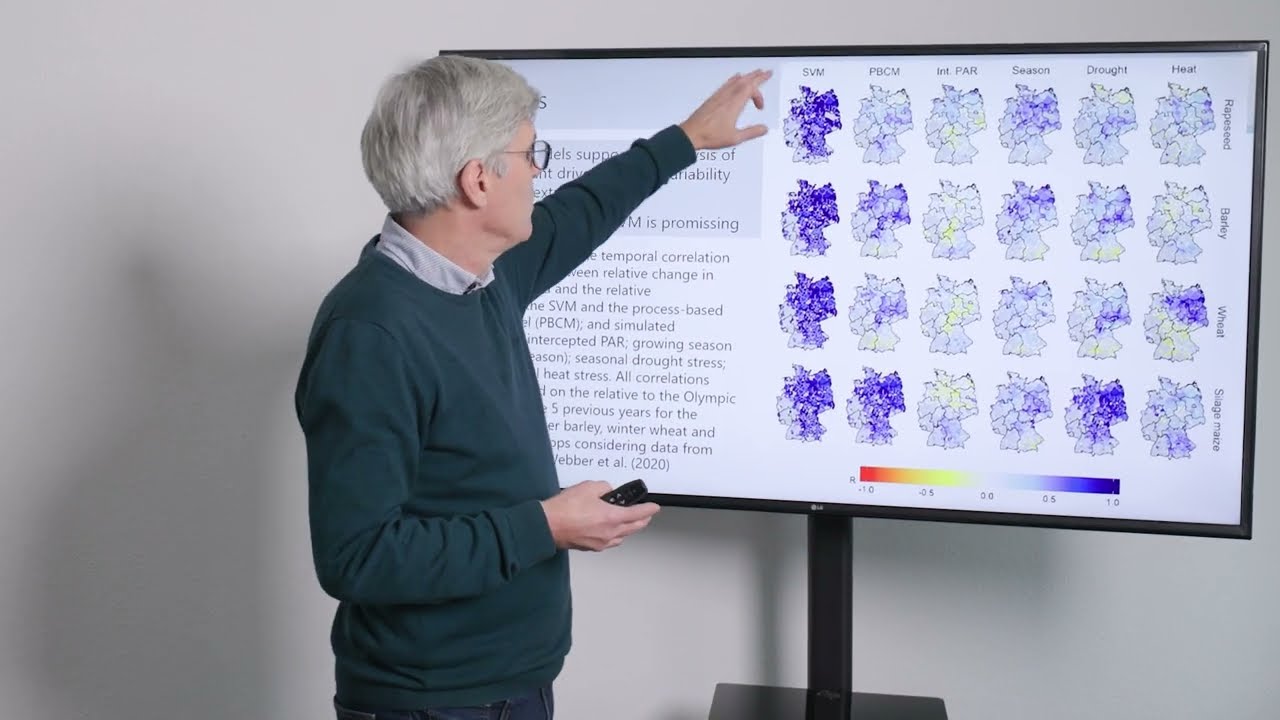
Estimating yield responses to climate variability and extreme events
Prof. Dr. Frank Ewert is Professor and head of the Crop Science Group, Institute of Crop Science and Resource Conservation (INRES) University of Bonn and Scientific Director of Leibniz Centre for Agricultural Landscape Research (ZALF) Webber H, Lischeid G, Sommer M, Finger R, Nendel C, Gaiser T, Ewert F. 2020. No perfect storm for crop yield failure in Germany. Environmental Research Letters 15 (10): 104012. doi: https://iopscience.iop.org/article/10.1088/1748-9326/aba2a4 Lischeid G, Webber H, Sommer M, Nendel C, Ewert F. 2022. Machine learning in crop yield modelling: A powerful tool, but no surrogate for science. Agricultural and Forest Meteorology 312, 108698. doi: https://doi.org/10.1016/j.agrformet.2021.108698
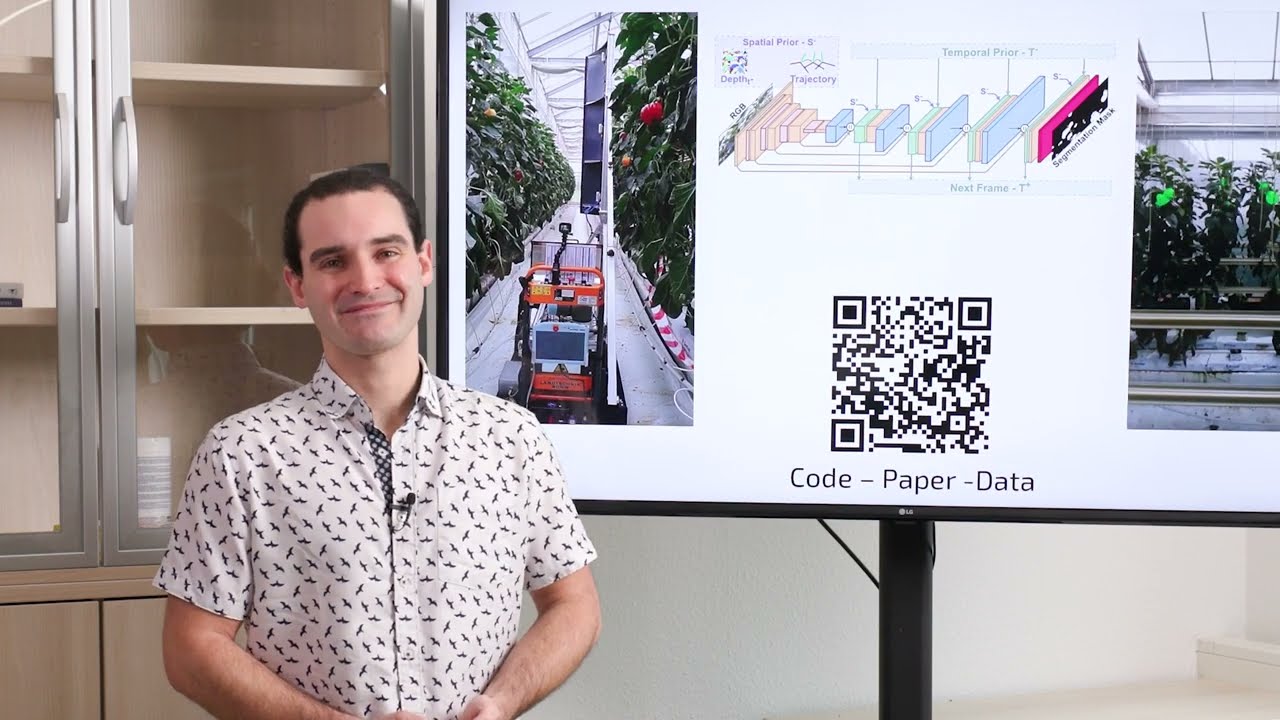
Explicitly Incorporating Spatial Information to Recurrent Networks for Agriculture
Claus Smitt is PhD Student at the Agricultural Robotics & Engineering department of the University of Bonn. Smitt, C., Halstead, M., Ahmadi, A., and McCool, C. , “Explicitly incorporating spatial information to recurrent networks for agriculture”. IEEE Robotics and Automation Letters, 7(4), 10017-10024. doi: 10.48550/arXiv.2206.13406
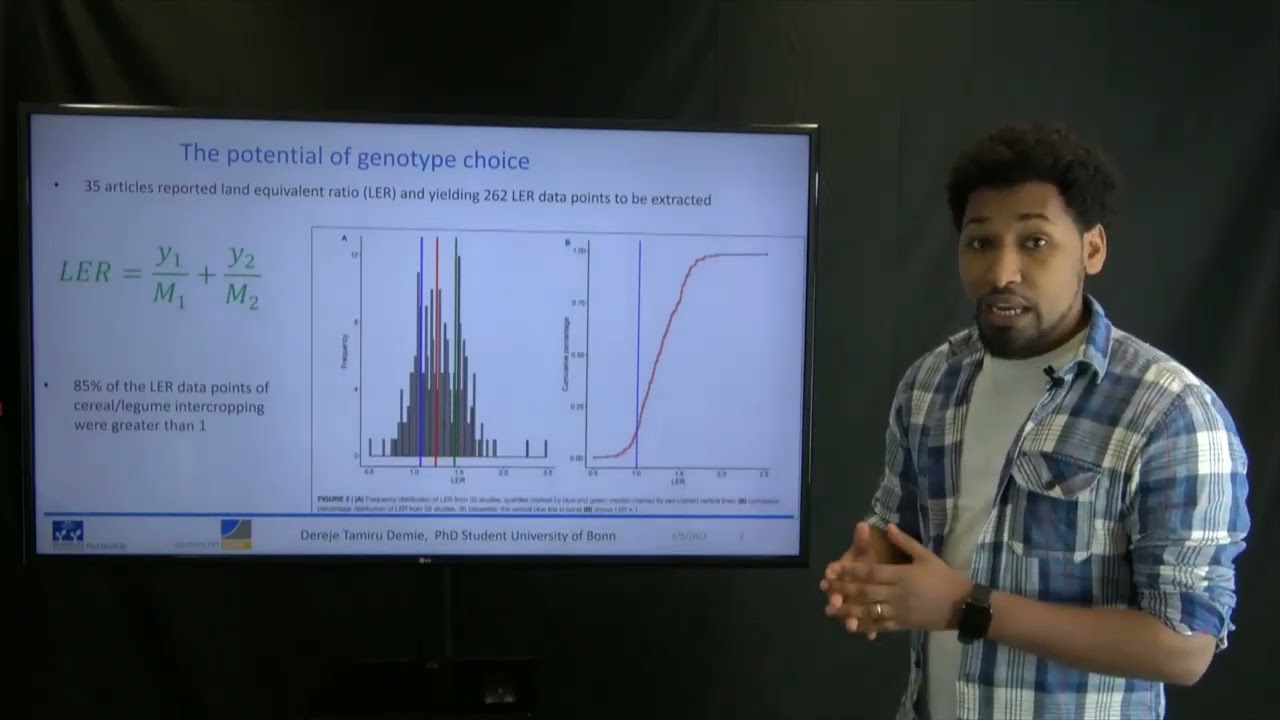
Mixture X Genotype Effects in Cereal/Legume Intercropping by Demie et al.
This short trailer is based on the following publication: D. Demie, T. Döring, M. Finckh, W. van der Werf, J. Enjalbert, and S. Seidel, “Mixture X Genotype Effects in Cereal/Legume Intercropping,” Frontiers in Plant Science, vol. 13, 2022. doi:10.3389/fpls.2022.846720 Full text available here: https://www.frontiersin.org/articles/10.3389/fpls.2022.846720/full
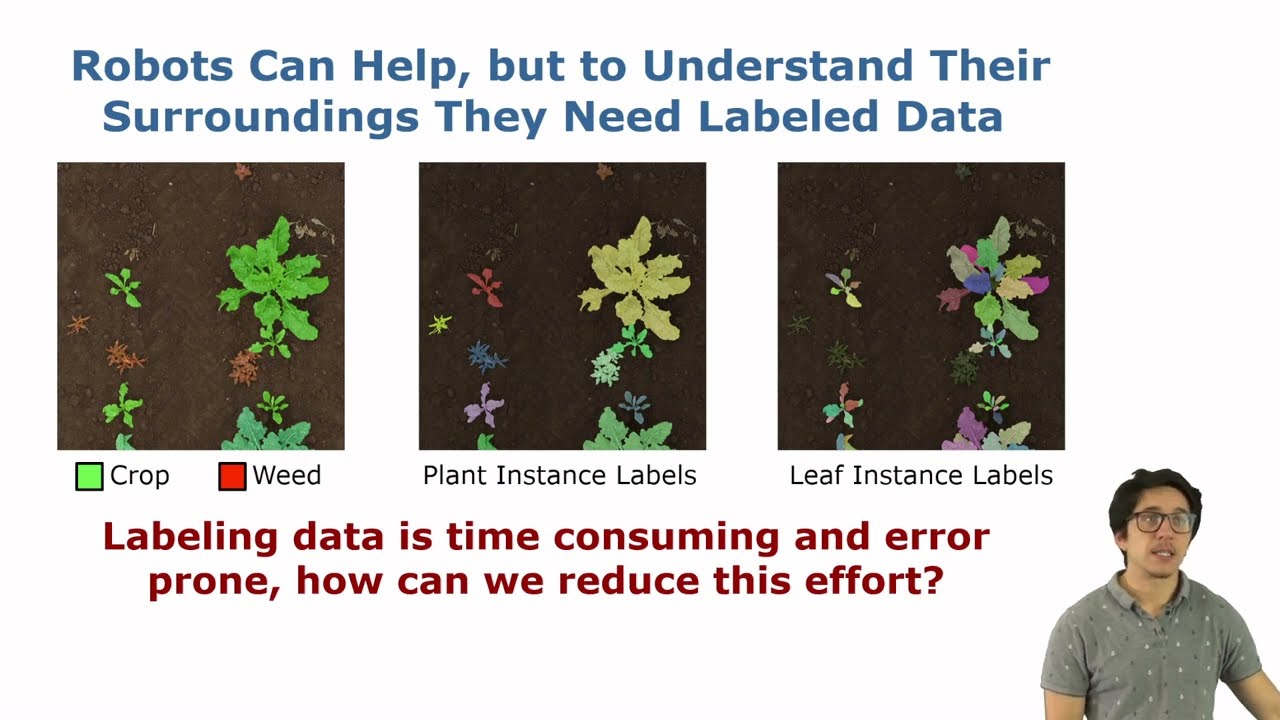
On Domain-Specific Pre-Training for Effective Semantic Perception in Agricult. Robotics (Roggiolani)
This short trailer is based on the following publication: G. Roggiolani, F. Magistri, T. Guadagnino, G. Grisetti, C. Stachniss, and J. Behley, “On Domain-Specific Pre-Training for Effective Semantic Perception in Agricultural Robotics,” Proceedings of the IEEE International Conference on Robotics & Automation (ICRA), 2023.
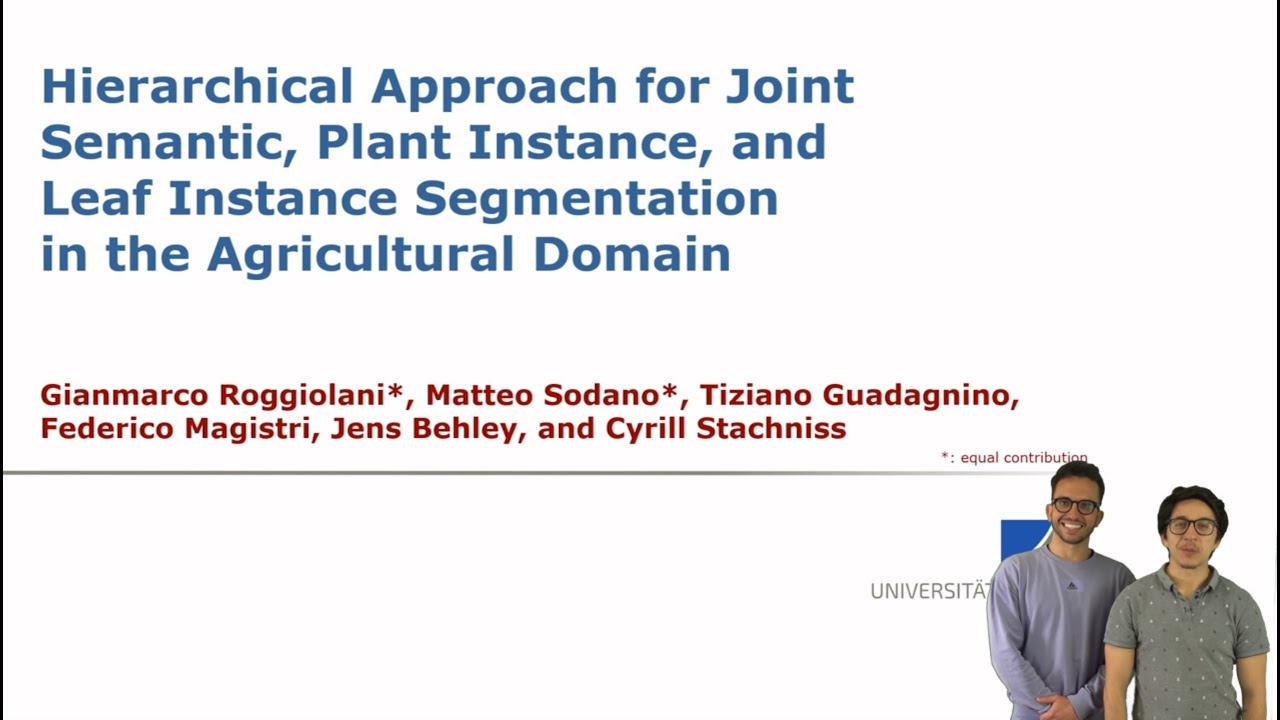
Hierarchical Approach for Joint Semantic, Plant & Leaf Instance Segmentation in the Agricult. Domain
This short trailer is based on the following publication: G. Roggiolani, M. Sodano, F. Magistri, T. Guadagnino, J. Behley, and C. Stachniss, “Hierarchical Approach for Joint Semantic, Plant Instance, and Leaf Instance Segmentation in the Agricultural Domain,” in Proceedings of the IEEE International Conference on Robotics & Automation (ICRA), 2023.
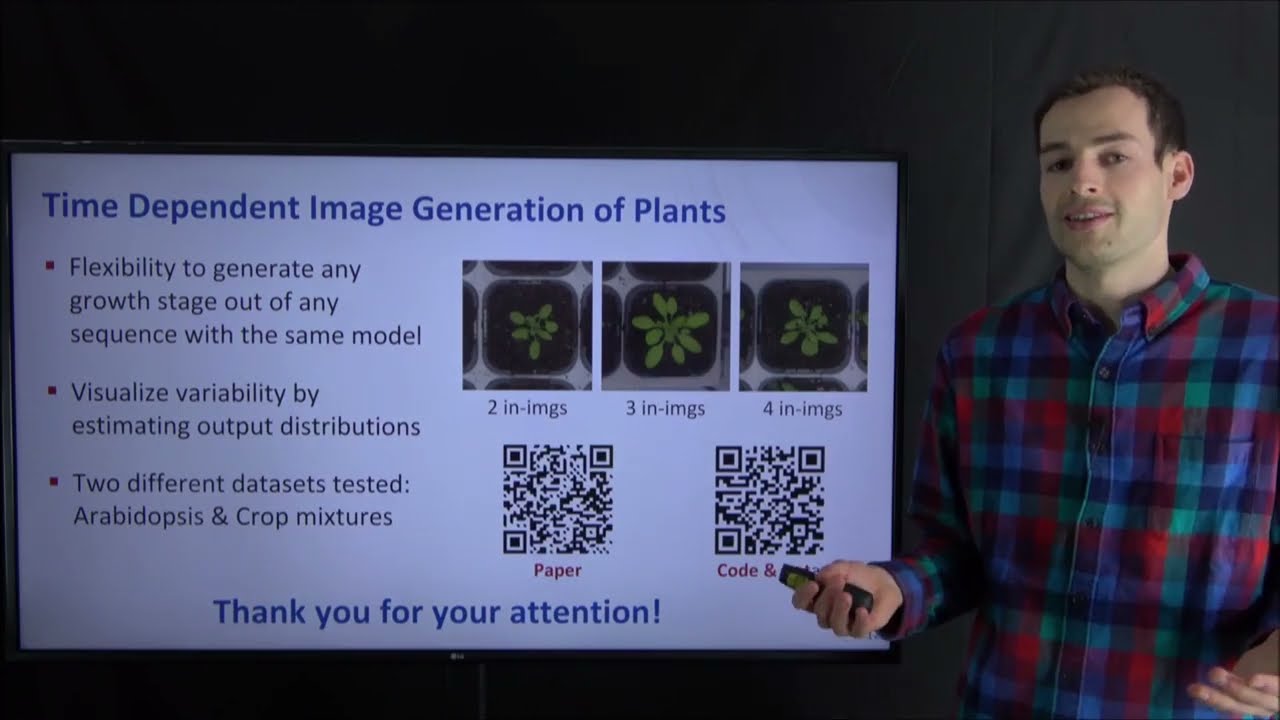
Time Dependent Image Generation of Plants from Incomplete Sequences with CNN-Transformer by L. Drees
This short trailer is based on the following publication: L. Drees, I. Weber, M. Russwurm, and R. Roscher, “Time Dependent Image Generation of Plants from Incomplete Sequences with CNN-Transformer,” in DAGM German Conference on Pattern Recognition , 2022, pp. 495-510. doi:https://doi.org/10.1007/978-3-031-16788-1_30 Full text available here: https://link.springer.com/chapter/10.1007/978-3-031-16788-1_30
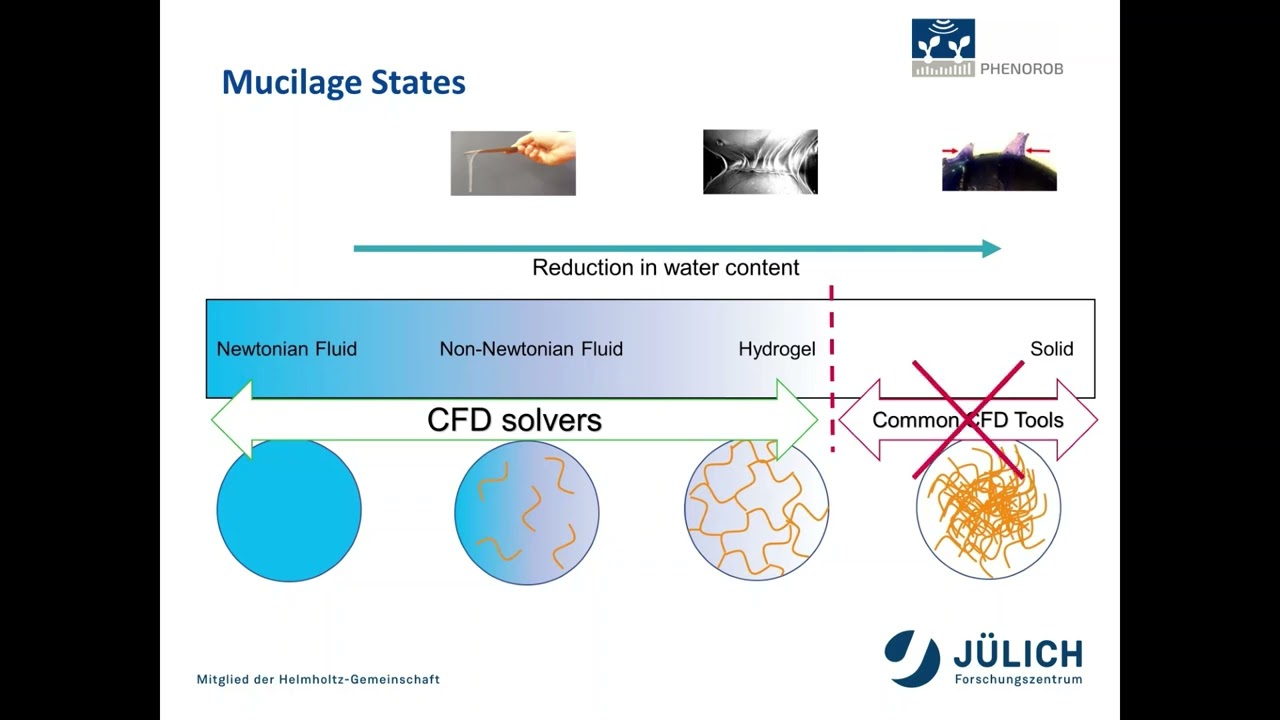
Pore-scale simulation of mucilage drainage by Omid Esmaeelipoor Jahromi et al.
This short trailer video is based on the following publication: O. Esmaeelipoor Jahromi, M. Knott, R. K. Janakiram, R. Rahim, and E. Kroener, “Pore-scale simulation of mucilage drainage,” Vadose Zone Journal, vol. e20218, pp. 1-13, 2022. doi:10.1002/vzj2.20218
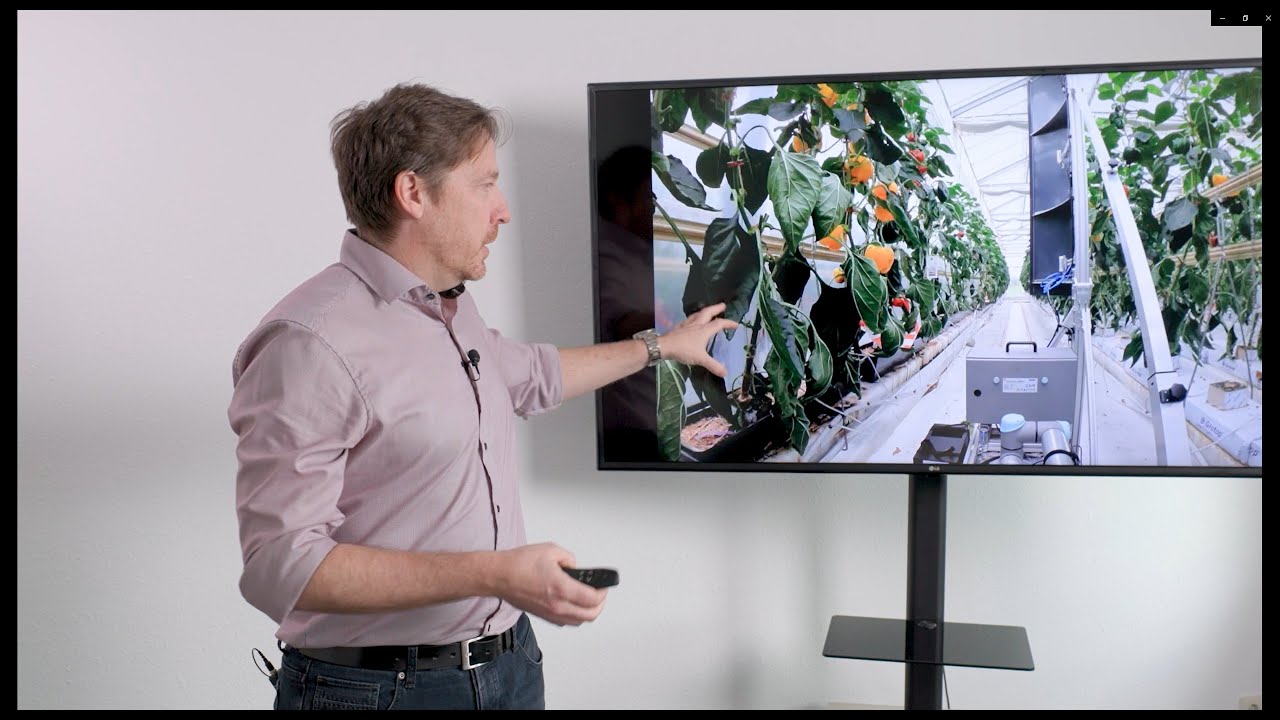
Crop Agnostic Monitoring Using Deep Learning
Prof. Dr. Chris McCool is Professor of Applied Computer Vision and Robotic Vision, head of the Agricultural Robotics and Engineering department at the University of Bonn M. Halstead, A. Ahmadi, C. Smitt, O. Schmittmann, C. McCool, “Crop Agnostic Monitoring Driven by Deep Learning”, in Front. Plant Sci. 12:786702. doi: 10.3389/fpls.2021.786702
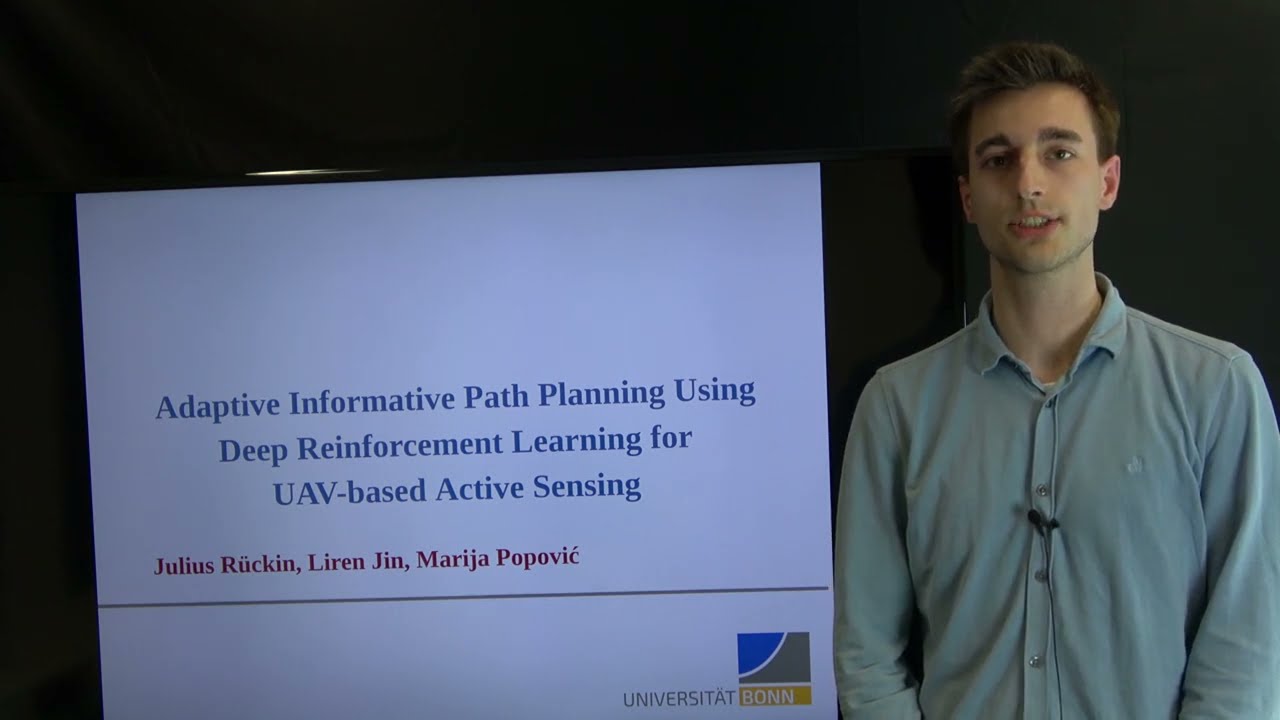
ICRA22: Adaptive Informative Path Planning Using Deep RL for UAV-based Active Sensing, Rückin et al.
Rückin J., Jin, L., and Popović, M., “Adaptive Informative Path Planning Using Deep RL for UAV-based Active Sensing,” in Proc. of the IEEE Intl. Conf. on Robotics & Automation (ICRA), 2022. doi: ICRA46639.2022.9812025 PDF: https://arxiv.org/abs/2109.13570 Code: https://github.com/dmar-bonn/ipp-rl
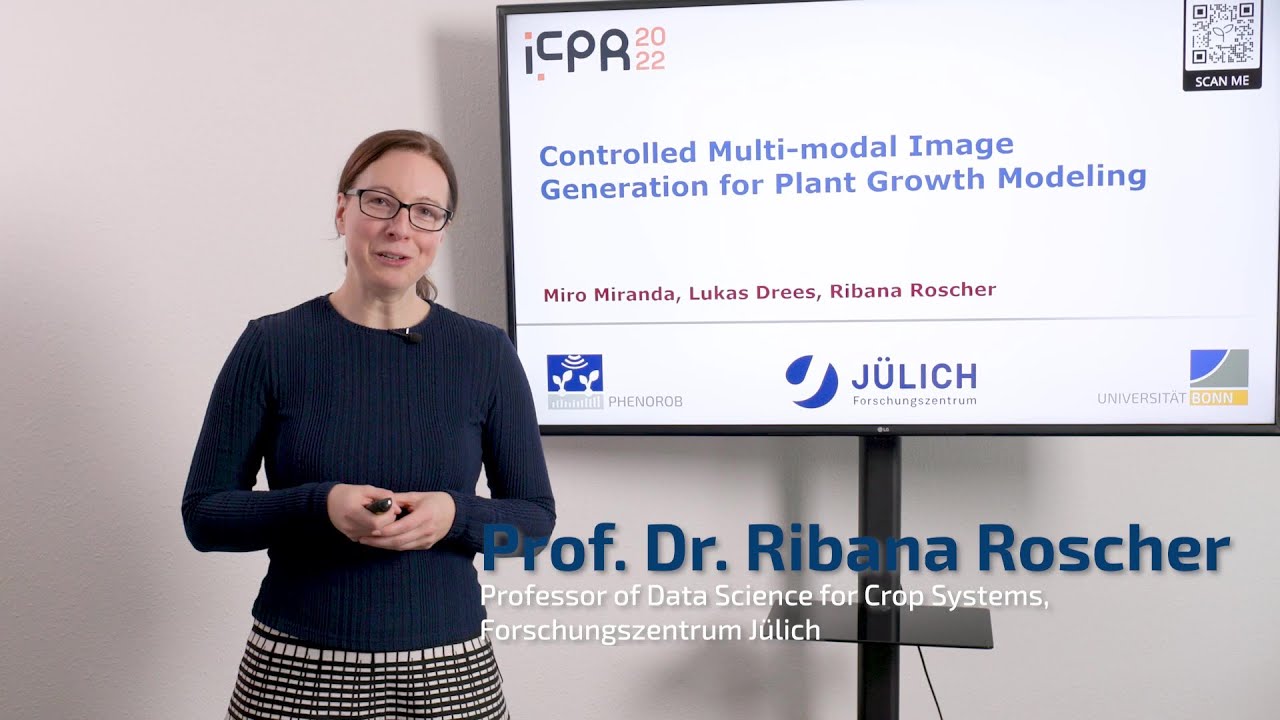
Controlled Multi-modal Image Generation for Plant Growth Modeling
Prof. Dr. Ribana Roscher is Professor of Data Science for Crop Systems, Institute of Bio- and Geosciences (IBG-2) at Forschungszentrum Jülich and Institute of Geodesy and Geoinformation (IGG), University of Bonn M. Miranda, L. Drees and R. Roscher, “Controlled Multi-modal Image Generation for Plant Growth Modeling,” in 2022 26th International Conference on Pattern Recognition (ICPR), Montreal, QC, Canada, 2022 pp. 5118-5124. doi: 10.1109/ICPR56361.2022.9956115
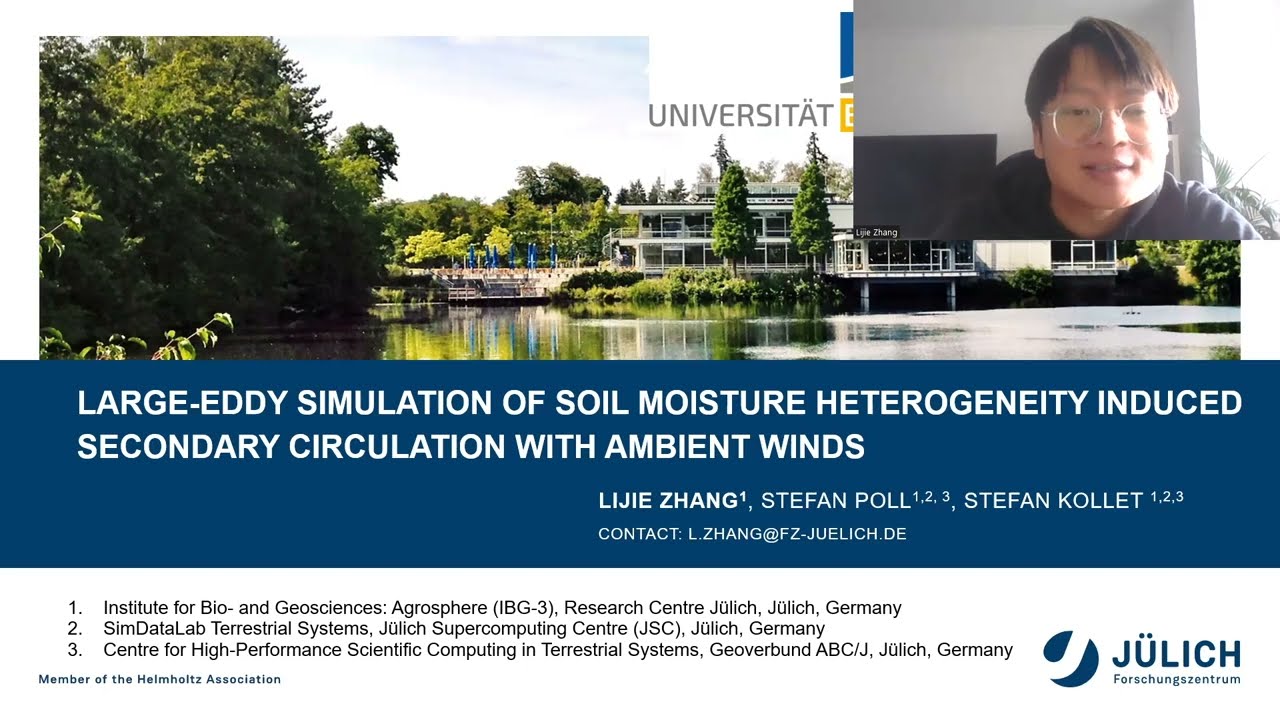
Large-eddy Simulation of Soil Moisture Heterogeneity Induced Secondary Circulation with Ambient Wind
This video is based on the following publication: L. Zhang, S. Poll, and S. Kollet, “Large-eddy Simulation of Soil Moisture Heterogeneity Induced Secondary Circulation with Ambient Winds,” Quarterly Journal of the Royal Meteorological Society, 2022. doi:10.5194/egusphere-egu22-5533
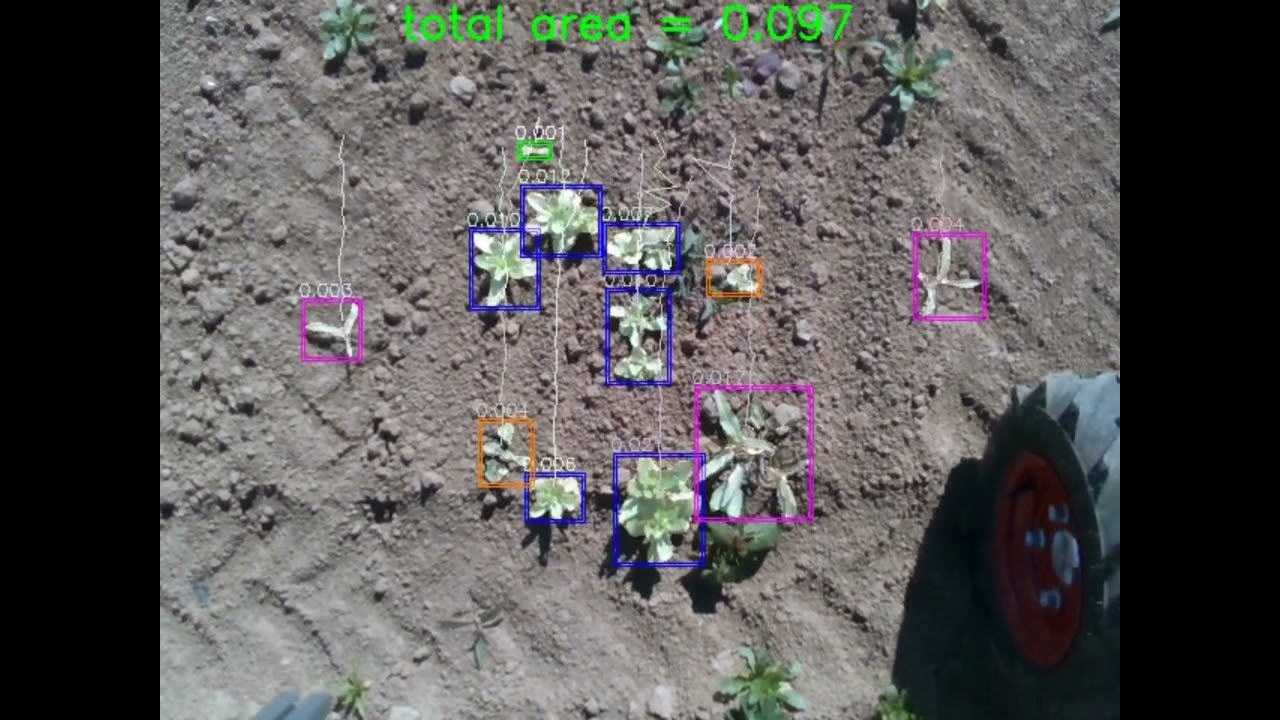
Crop and Weed Counting and Tracking
Example video from Crop Agnostic Monitoring Driven by Deep Learning available here: https://www.frontiersin.org/articles/10.3389/fpls.2021.786702/full?&utm_source=Email_to_authors_&utm_medium=Email&utm_content=T1_11.5e1_author&utm_campaign=Email_publication&field=&journalName=Frontiers_in_Plant_Science&id=786702 The different colours for the bounding boxes represent the species of plant. The outer box is the final prediction over the whole tracklet (most consistent class that is predicted) and the inner box is the class (species) for the current frame. In this example we have 8 classes of plants. The numbers for each plant are the visual surface area of the plant taken by using a stereo camera and the instance-based semantic segmentation. Citation: Halstead M, Ahmadi A, Smitt C, Schmittmann O and McCool C (2021) Crop Agnostic Monitoring Driven by Deep Learning. Front. Plant Sci. 12:786702. doi: 10.3389/fpls.2021.786702
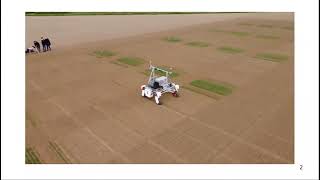
Explicitly Incorporating Spatial Information to Recurrent Networks for Agriculture
IROS 2022 Best Paper Award on Agri-Robotics – Sponsored by YANMAR “Explicitly Incorporating Spatial Information to Recurrent Networks for Agriculture,” by Claus Smitt, Michael Allan Halstead, Alireza Ahmadi, and Christopher Steven McCool from University of Bonn. Read more: https://events.infovaya.com/presentation?id=86839 More robot videos from IROS: https://spectrum.ieee.org/robot-videos-iros-award-winners #ieee #engineering #robotics

Hypermap Mapping Framework and its Application to Autonomous Semantic Exploration by T Zaenker et al
This paper trailer video is based on the following publication: T. Zaenker, F. Verdoja, and V. Kyrki, “Hypermap Mapping Framework and its Application to Autonomous Semantic Exploration,” in 2020 IEEE Conference on Multisensor Fusion and Integration, 2020. To learn more, check out the full publication here: https://www.semanticscholar.org/paper/Hypermap-Mapping-Framework-and-its-Application-to-Zaenker-Verdoja/36aa4db3bd911b0824fdf1343900521f214c841c
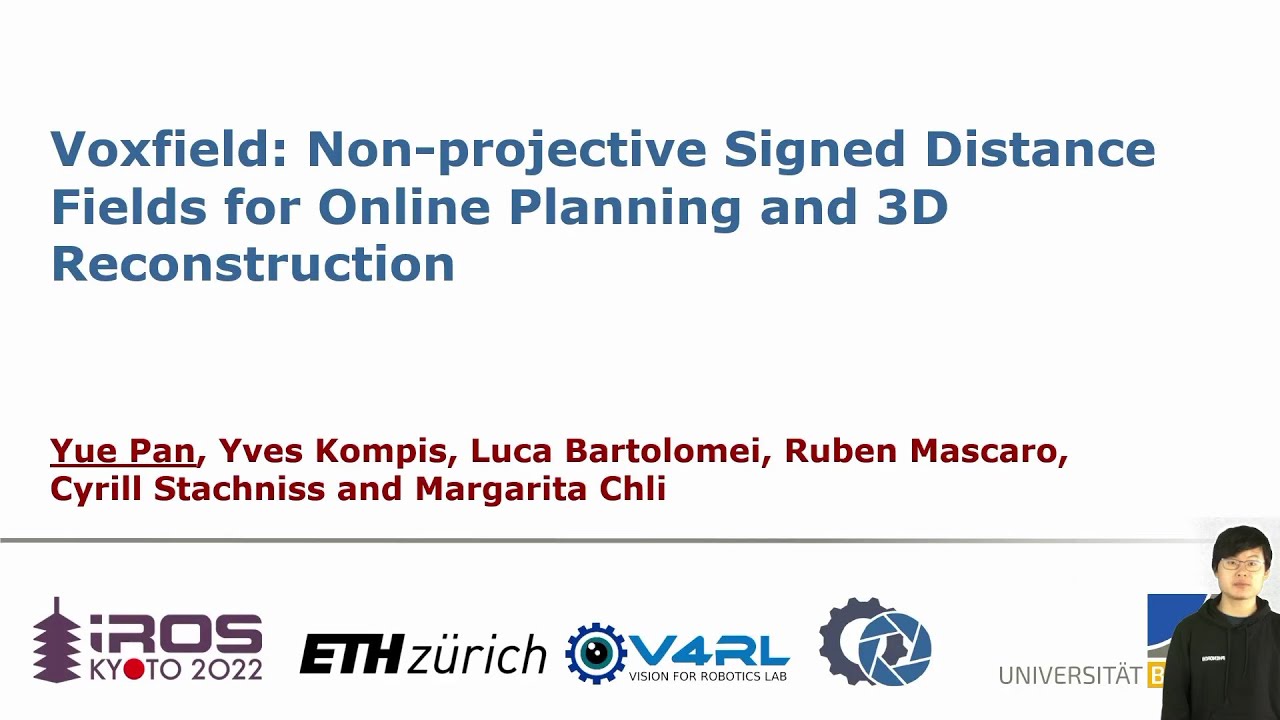
Talk by Y. Pan: Voxfield: Non-Projective Signed Distance Fields (IROS’22)
IROS 2022 Talk by Yue Pan about the paper: Y. Pan, Y. Kompis, L. Bartolomei, R. Mascaro, C. Stachniss, and M. Chli, “Voxfield: Non-Projective Signed Distance Fields for Online Planning and 3D Reconstruction,” in Proc. of the IEEE/RSJ Int. Conf. on Intelligent Robots and Systems (IROS), 2022. Paper: https://www.ipb.uni-bonn.de/wp-content/papercite-data/pdf/pan2022iros.pdf Code: https://github.com/VIS4ROB-lab/voxfield
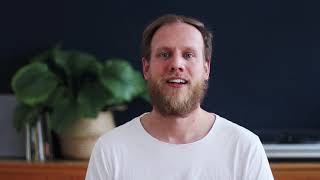
C. Pahmeyer, T. Kuhn & W. Britz – ‘Fruchtfolge’: A crop rotation decision support system (Trailer)
Watch the full presentation: http://digicrop.de/program/fruchtfolge-a-crop-rotation-decision-support-system-for-optimizing-cropping-choices-with-big-data-and-spatially-explicit-modeling/
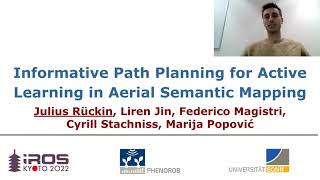
Talk by J. Rückin: Informative Path Planning for Active Learning in Aerial Semantic Map… (IROS’22)
IROS 2020 talk by Julius Rückin about the paper J. Rückin, L. Jin, F. Magistri, C. Stachniss, and M. Popović, “Informative Path Planning for Active Learning in Aerial Semantic Mapping,” in Proc. of the IEEE/RSJ Int. Conf. on Intelligent Robots and Systems (IROS), 2022. PDF: https://www.ipb.uni-bonn.de/wp-content/papercite-data/pdf/rueckin2022iros.pdf

Talk by F. Magistri: 3D Shape Completion and Reconstruction for Agricultural Robots (RAL-IROS’22)
IROS 2020 Talk by Federico Magistri on F. Magistri, E. Marks, S. Nagulavancha, I. Vizzo, T. Läbe, J. Behley, M. Halstead, C. McCool, and C. Stachniss, “Contrastive 3D Shape Completion and Reconstruction for Agricultural Robots using RGB-D Frames,” IEEE Robotics and Automation Letters (RA-L), vol. 7, iss. 4, pp. 10120-10127, 2022. Paper: https://www.ipb.uni-bonn.de/wp-content/papercite-data/pdf/magistri2022ral-iros.pdf

Innovation context & technology traits explain heterogeneity across studies of agri. tech. adoption
This PhenoRob paper trailer is based on the following publication: Schulz, Dario & Börner, Jan, “Innovation context and technology traits explain heterogeneity across studies of agricultural technology adoption: A meta-analysis,” Journal of Agricultural Economics, 2022. DOI: 10.1111/1477-9552.12521.
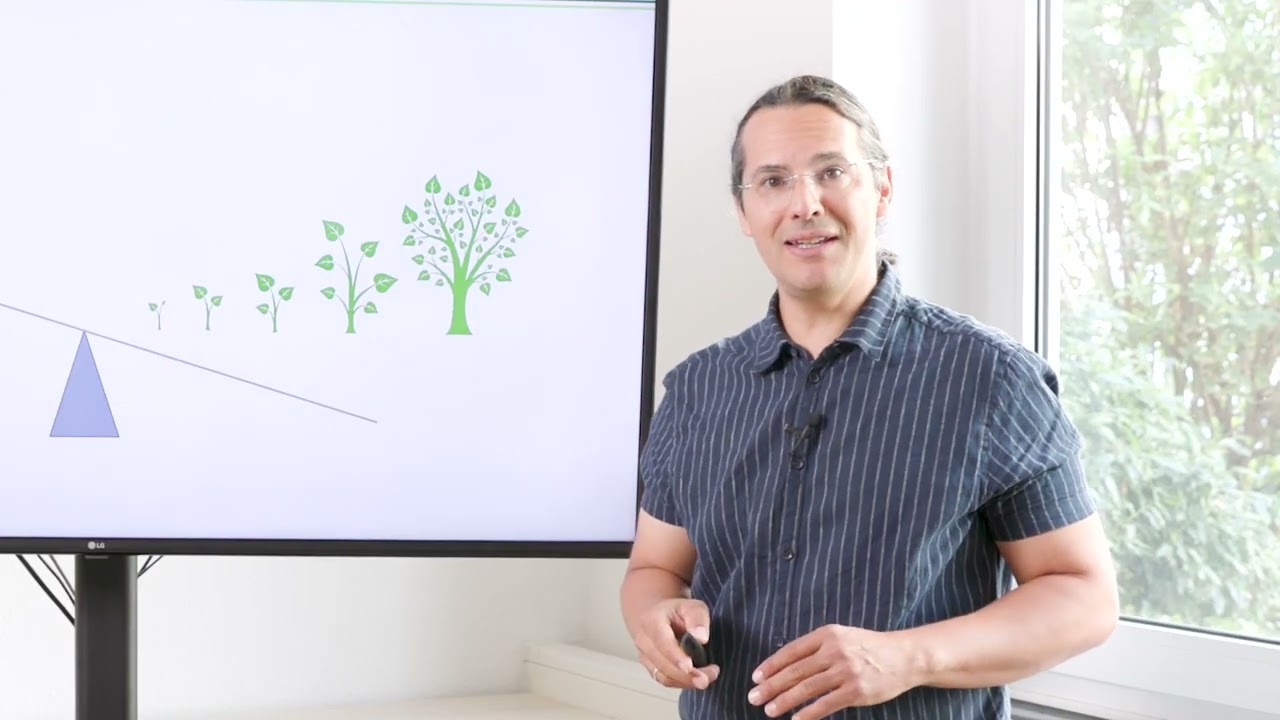
Djamei: Many ways to TOPLESS– manipulation of plant auxin signaling by a cluster of fungal effectors
Prof. Dr. Armin Djamei is Professor of Plant Pathology, Institute of Crop Science and Resource Conservation (INRES) at the University of Bonn. Bindics, J., Khan, M., Uhse, S., Kogelmann, B., Baggely, L., Reumann, D., Ingole, K.D., Stirnberg, A., Rybecky, A., Darino, M., Navarrete, F., Doehlemann, G. and Djamei, A. (2022), Many ways to TOPLESS – manipulation of plant auxin signalling by a cluster of fungal effectors. New Phytologist. https://doi.org/10.1111/nph.18315
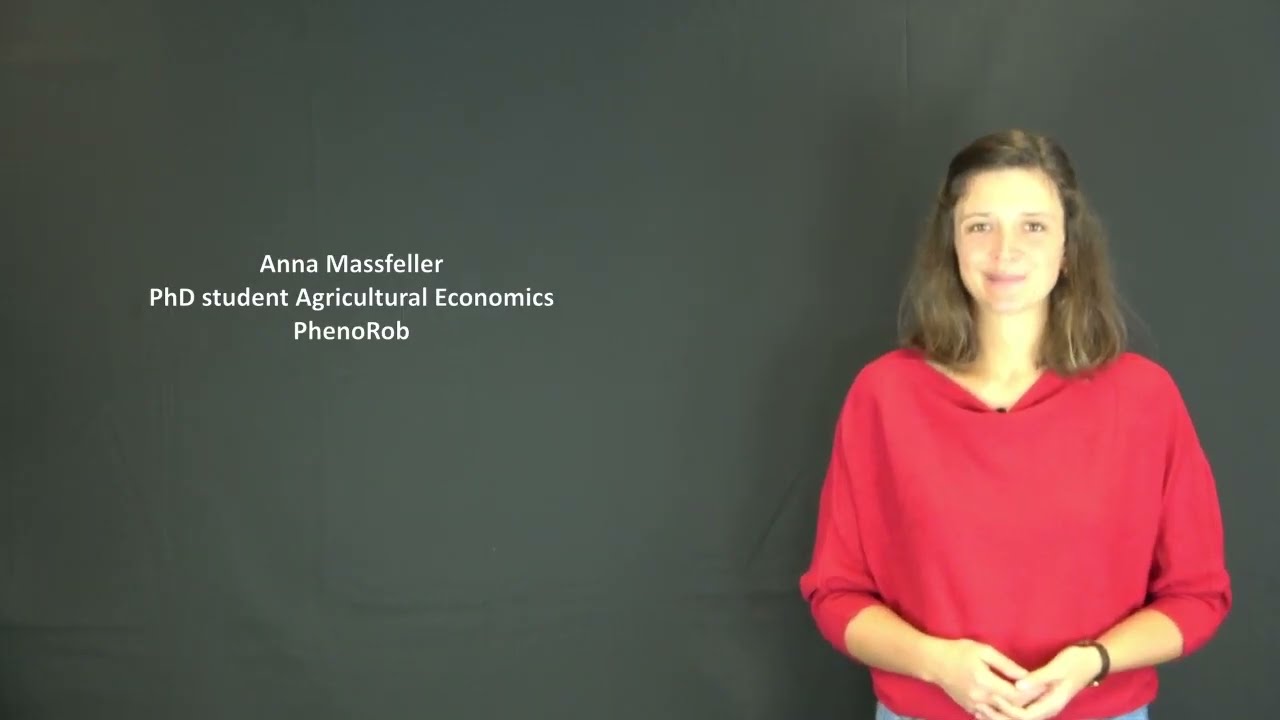
Farmers’ acceptance of results-based agri-environmental schemes: A German perspective
This PhenoRob paper trailer is based on the following publication: A. Massfeller, M. Meraner, S. Huettel, and R. Uehleke, “Farmers’ acceptance of results-based agri-environmental schemes: A German perspective,” Land Use Policy, vol. 120, 2022. doi:10.1016/j.landusepol.2022.106281
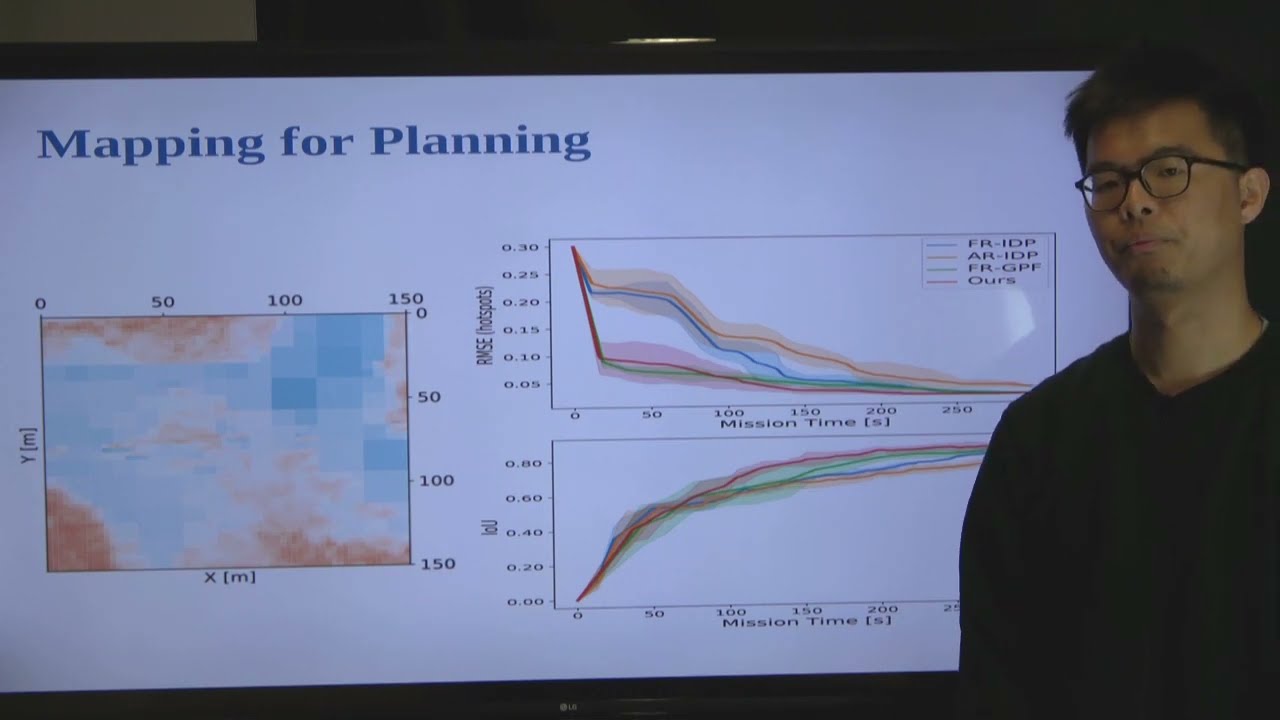
Adaptive-Resolution Field Mapping Using Gaussian Process Fusion With Integral Kernels by L.Jin et al
This short paper trailer is based on the following publication: L. Jin, J. Rückin, S. H. Kiss, T. Vidal-Calleja, and M. Popović, “Adaptive-Resolution Field Mapping Using Gaussian Process Fusion With Integral Kernels,” IEEE Robotics and Automation Letters, vol. 7, pp. 7471-7478, 2022. doi:10.1109/LRA.2022.3183797
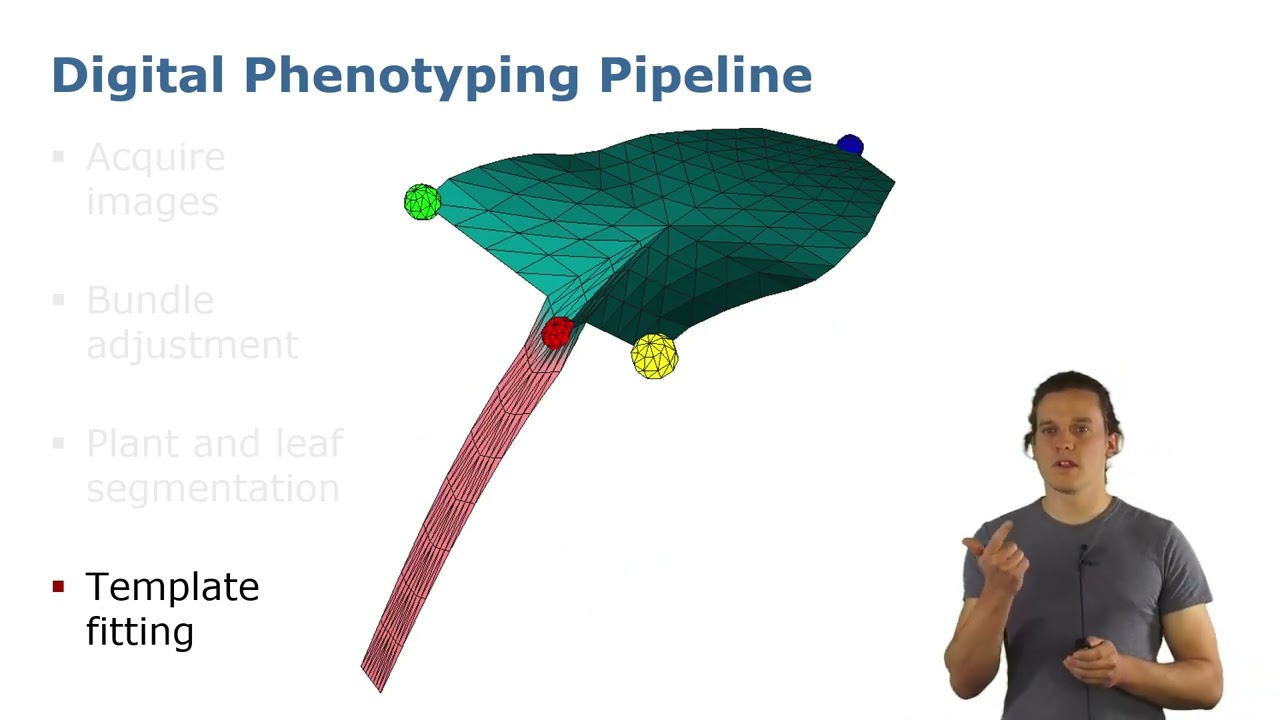
ICRA’22: Precise 3D Reconstruction of Plants from UAV Imagery … by Marks et al.
E. Marks, F. Magistri, and C. Stachniss, “Precise 3D Reconstruction of Plants from UAV Imagery Combining Bundle Adjustment and Template Matching,” in Proc.~of the IEEE Intl.~Conf.~on Robotics & Automation (ICRA), 2022. PDF: https://www.ipb.uni-bonn.de/wp-content/papercite-data/pdf/marks2022icra.pdf #UniBonn #StachnissLab #robotics
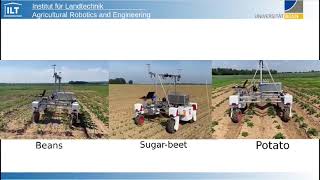
Towards Autonomous Visual Navigation in Arable Fields
Rou pare can be found in Arxiv at: [Towards Autonomous Crop-Agnostic Visual Navigation in Arable Fields](https://arxiv.org/abs/2109.11936) You can find the implementation in : [visual-multi-crop-row-navigation](https://github.com/Agricultural-Robotics-Bonn/visual-multi-crop-row-navigation) more detail about our project BonnBot-I and Phenorob at: https://www.phenorob.de/ http://agrobotics.uni-bonn.de/
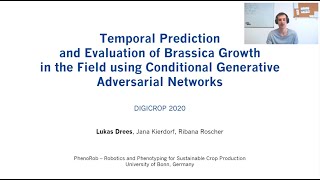
Lukas Drees – Temporal Prediction & Evaluation of Brassica Growth in the Field using cGANs (Trailer)
Watch the full presentation: http://digicrop.de/program/temporal-prediction-and-evaluation-of-brassica-growth-in-the-field-using-conditional-generative-adversarial-networks/
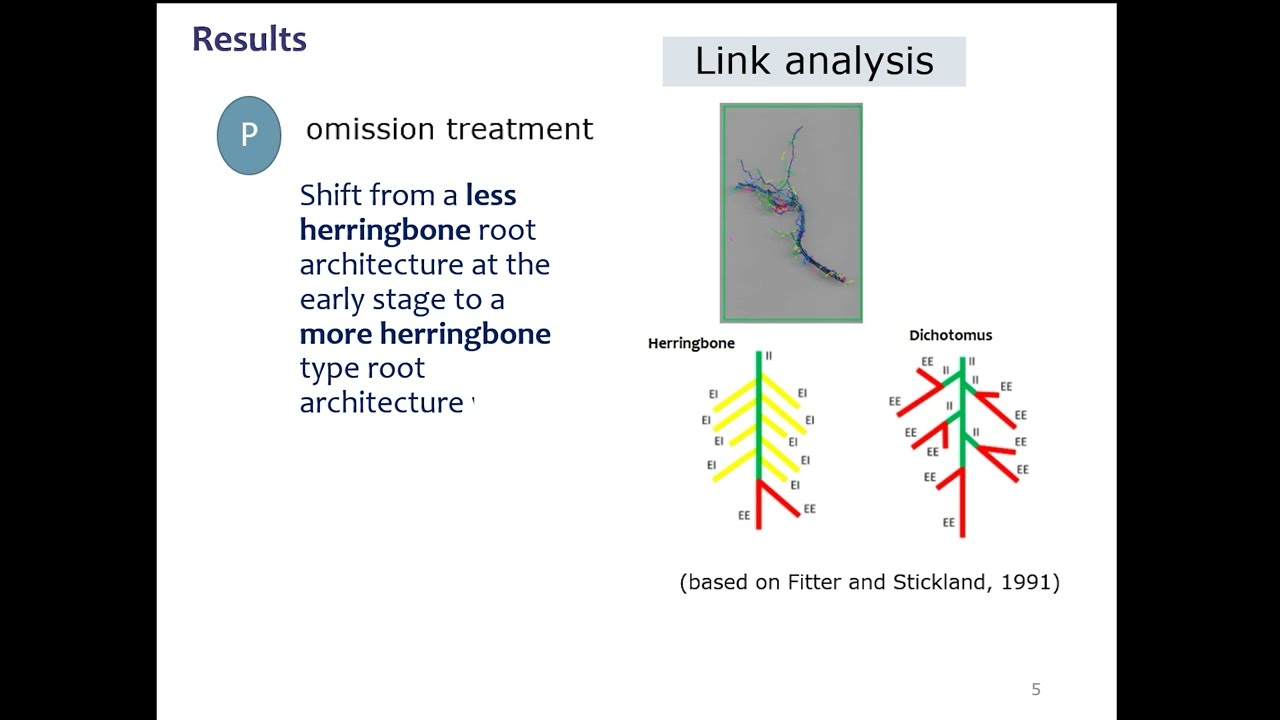
Sugar Beet Shoot and Root Phenotypic Plasticity by S. Hadir et al.
This short paper trailer video is based on the following publication: S. Hadir, T. Gaiser, H. Hüging, M. Athmann, D. Pfarr, R. Kemper, F. Ewert, and S. Seidel, “Sugar Beet Shoot and Root Phenotypic Plasticity to Nitrogen, Phosphorus, Potassium and Lime Omission,” Agriculture, vol. 11, iss. 1, 2021. Find more info here: https://www.mdpi.com/2077-0472/11/1/21
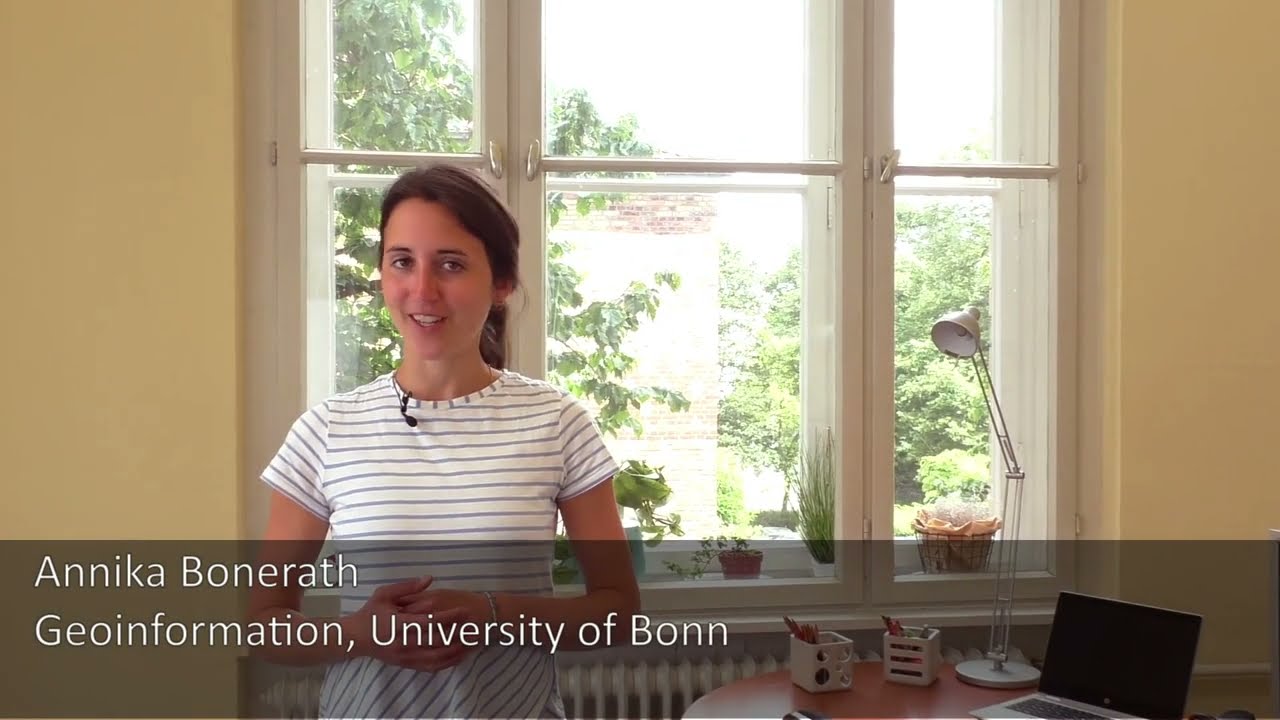
Shortcut Hulls: Vertex-restricted Outer Simplifications of Polygons by A. Bonerath et al.
This short paper trailer video is based on the following publication: A. Bonerath, J. Haunert, J. S. B. Mitchell, and B. Niedermann, “Shortcut Hulls: Vertex-restricted Outer Simplifications of Polygons,” in Proceedings of the 33rd Canadian Conference on Computational Geometry , 2021, pp. 12-23.
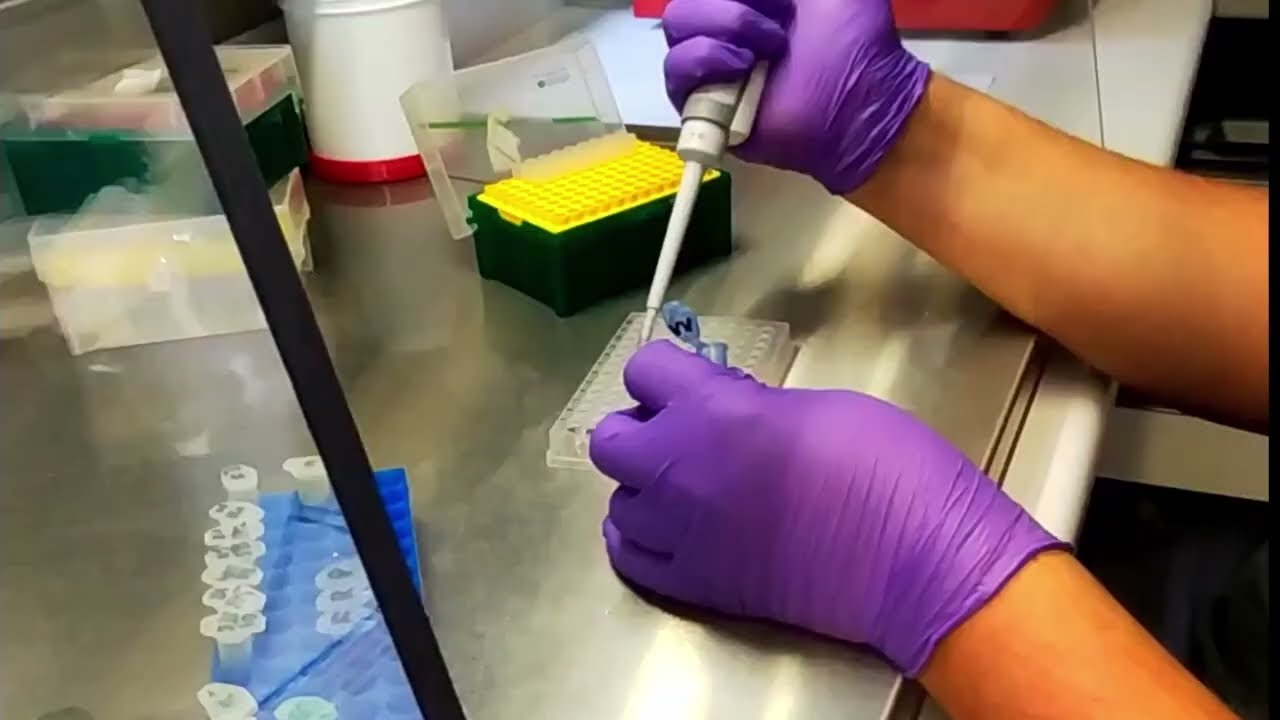
Cercospora leaf spot modeling in sugar beet by Ispizua, Barreto, Günder, Bauckhage & Mahlein
This short paper trailer video is based on the following publication: F. R. Ispizua Yamati, A. Barreto, A. Günder, C. Bauckhage, and A. -K. Mahlein, “Sensing the occurrence and dynamics of Cercospora leaf spot disease using UAV-supported image data and deep learning,” Sugar Industry, vol. 147, iss. 2, pp. 79-86, 2022. Find more info here: https://sugarindustry.info/paper/28345/ https://www.researchgate.net/publication/358243320_Sensing_the_occurrence_and_dynamics_of_Cercospora_leaf_spot_disease_using_UAV-supported_image_data_and_deep_learning

LatticeNet: fast spatio-temporal point cloud segmentation using permutohedral lattices (Rosu et al.)
This short paper trailer video is based on the following publication: R. A. Rosu, P. Schütt, J. Quenzel, and S. Behnke, “LatticeNet: fast spatio-temporal point cloud segmentation using permutohedral lattices,” Autonomous Robots, p. 1-16, 2021.

EasyPBR: A Lightweight Physically-Based Renderer
Presentation for paper by Radu Alexandru Rosu and Sven Behnke: “EasyPBR: A Lightweight Physically-Based Renderer” 16th International Conference on Computer Graphics Theory and Applications (GRAPP), 2021 Modern rendering libraries provide unprecedented realism, producing real-time photorealistic 3D graphics on commodity hardware. Visual fidelity, however, comes at the cost of increased complexity and difficulty of usage, with many rendering parameters requiring a deep understanding of the pipeline. We propose EasyPBR as an alternative rendering library that strikes a balance between ease-of-use and visual quality. EasyPBR consists of a deferred renderer that implements recent state-of-the-art approaches in physically based rendering. It offers an easy-to-use Python and C++ interface that allows high-quality images to be created in only a few lines of code or directly through a graphical user interface. The user can choose between fully controlling the rendering pipeline or letting EasyPBR automatically infer the best parameters based on the current scene composition. The EasyPBR library can help the community to more easily leverage the power of current GPUs to create realistic images. These can then be used as synthetic data for deep learning or for creating animations for academic purposes. http://www.ais.uni-bonn.de/papers/GRAPP_2021_Rosu_EasyPBR.pdf
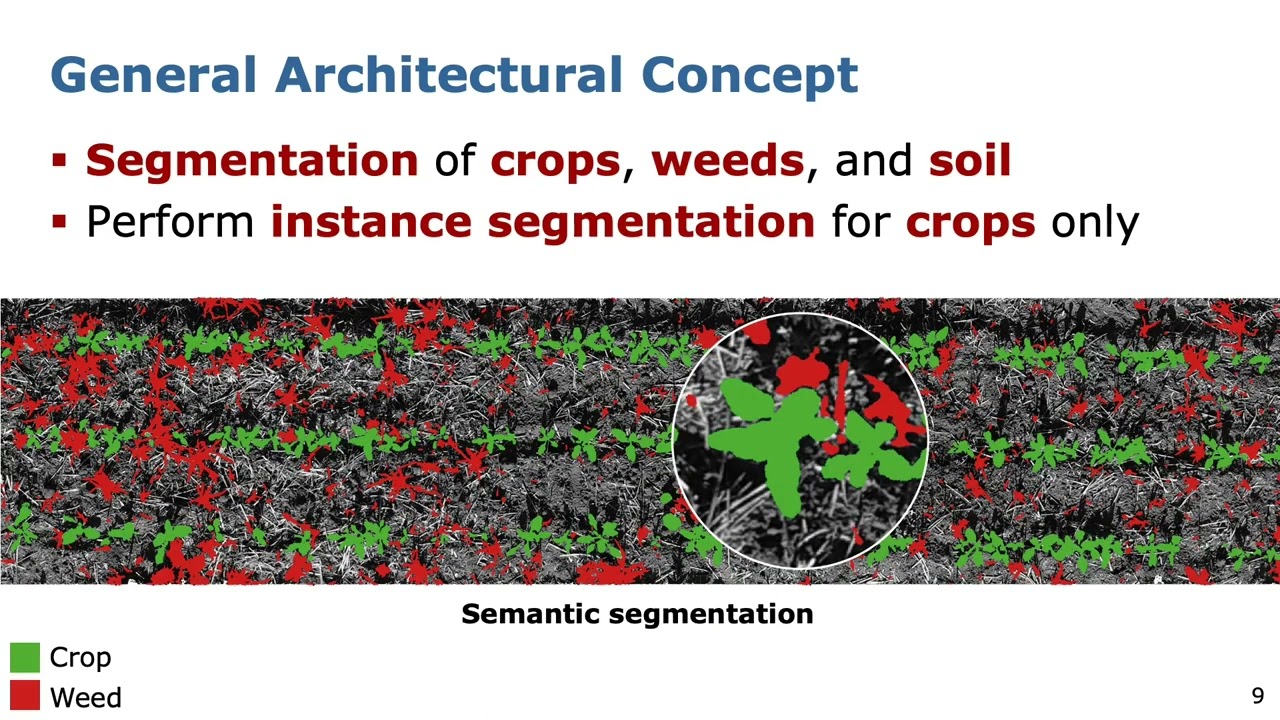
RAL-ICRA’22: Joint Plant and Leaf Instance Segmentation on Field-Scale UAV Imagery by Weyler et al.
J. Weyler, J. Quakernack, P. Lottes, J. Behley, and C. Stachniss, “Joint Plant and Leaf Instance Segmentation on Field-Scale UAV Imagery,” IEEE Robotics and Automation Letters (RA-L), vol. 7, iss. 2, pp. 3787-3794, 2022. doi:10.1109/LRA.2022.3147462 PDF: https://www.ipb.uni-bonn.de/wp-content/papercite-data/pdf/weyler2022ral.pdf #UniBonn #StachnissLab #robotics
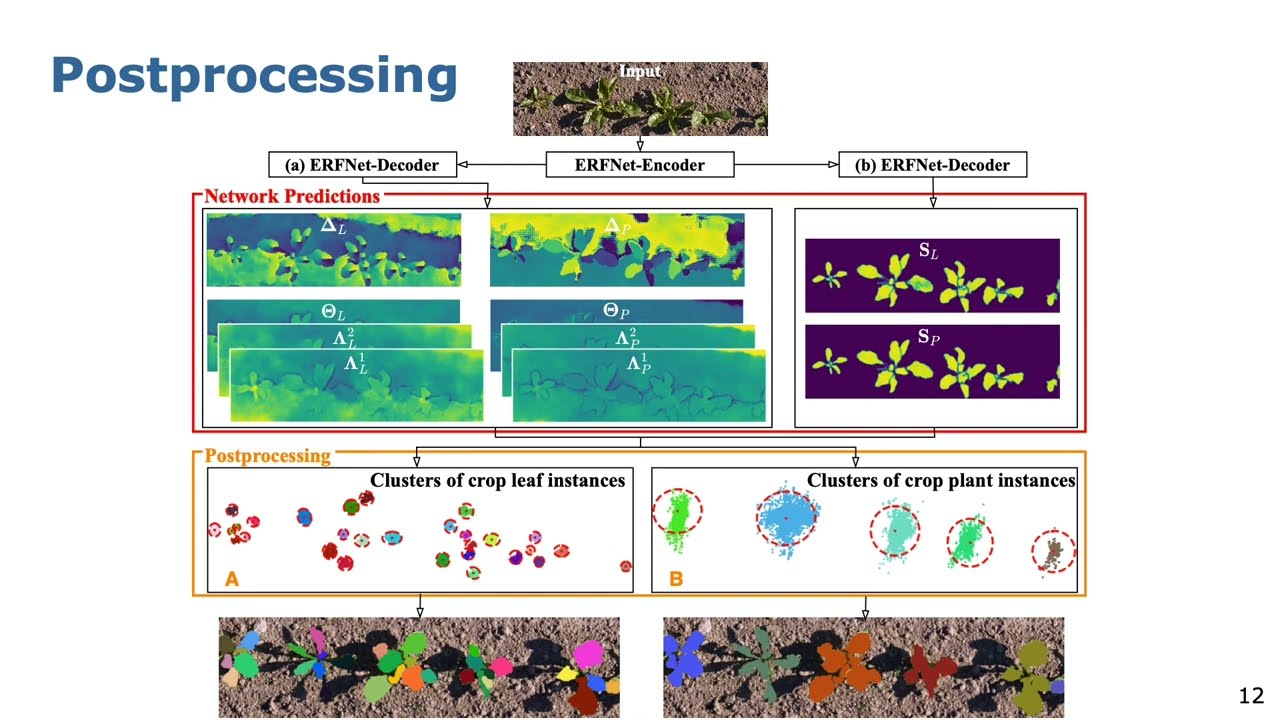
WACV’22: In-Field Phenotyping Based on Crop Leaf and Plant Instance Segmentation by Weyler et al.
J. Weyler, F. and Magistri, P. Seitz, J. Behley, and C. Stachniss, “In-Field Phenotyping Based on Crop Leaf and Plant Instance Segmentation,” in Proc. of the Winter Conf. on Applications of Computer Vision (WACV), 2022. PDF: https://www.ipb.uni-bonn.de/wp-content/papercite-data/pdf/weyler2022wacv.pdf #UniBonn #StachnissLab #robotics

ICRA’21: Phenotyping Exploiting Differentiable Rendering with Consistency Loss by Magistri et al.
F. Magistri, N. Chebrolu, J. Behley, and C. Stachniss, “Towards In-Field Phenotyping Exploiting Differentiable Rendering with Self-Consistency Loss,” in Proceedings of the IEEE Int. Conf. on Robotics & Automation (ICRA), 2021. Paper: https://www.ipb.uni-bonn.de/wp-content/papercite-data/pdf/magistri2021icra.pdf #UniBonn #StachnissLab #robotics #PhenoRob #neuralnetworks #talk
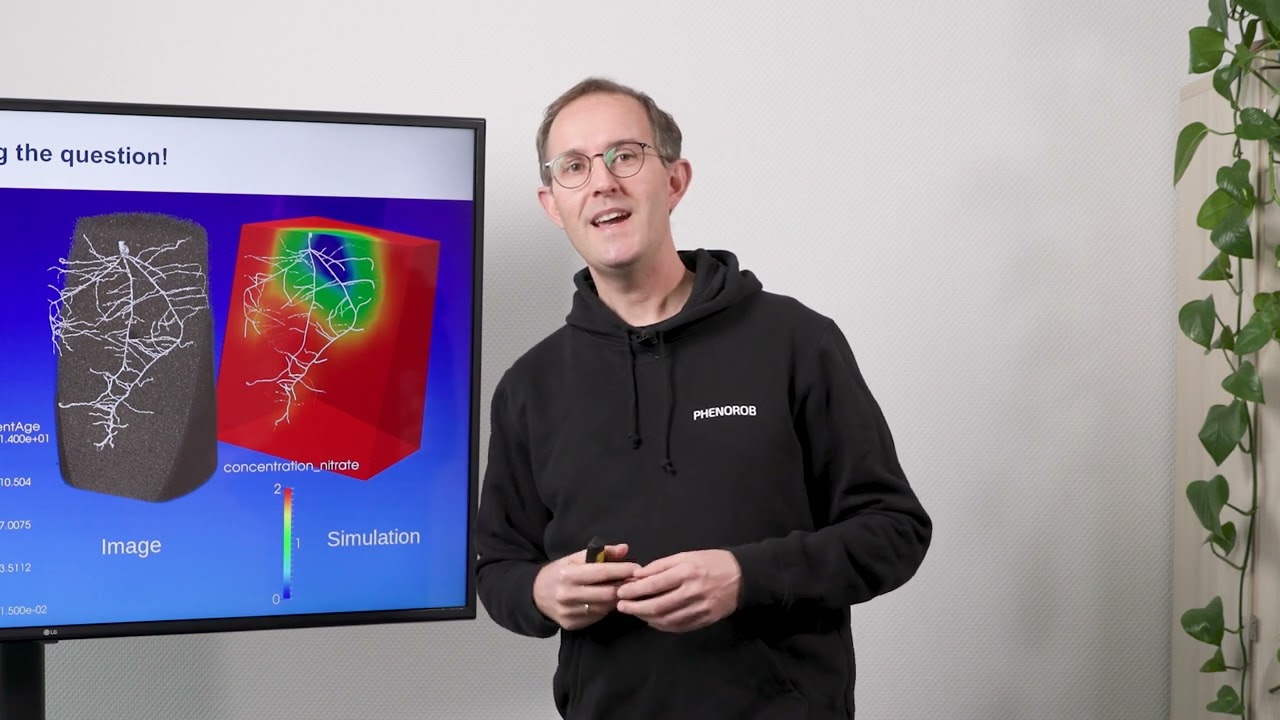
Johannes Postma: Crop Improvement From Phenotyping Roots – Highlights Reveal Expanding Opportunities
Johannes A. Postma is a PhenoRob Member and Researcher at the Institute of Bio- and Geosciences (IBG-2), Forschungszentrum Jülich Saoirse R. Tracy, Kerstin A. Nagel, Johannes A. Postma, Heike Fassbender, Anton Wasson, Michelle Watt (2020), Crop Improvement from Phenotyping Roots: Highlights Reveal Expanding Opportunities, Trends in Plant Science, Volume 25, Issue 1, Pages 105-118 https://doi.org/10.1016/j.tplants.2019.10.015
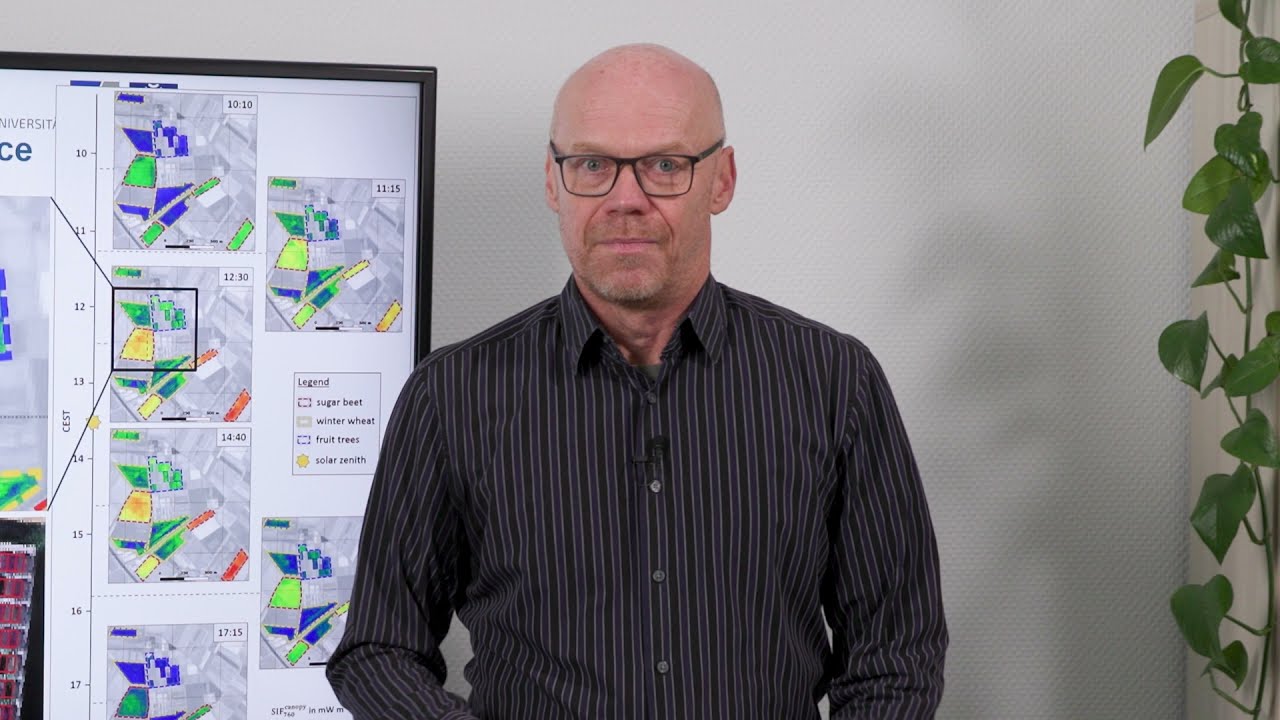
Uwe Rascher: Measuring and understanding the dynamics of plant photosynthesis across scales…
Measuring and understanding the dynamics of plant photosynthesis across scales – from single plants to satellites Prof. Dr. Uwe Rascher is Principal Investigator at PhenoRob and Professor of Quantitative Physiology of Crops, Institute of Bio- and Geosciences (IBG-2), Forschungszentrum Jülich and Institute of Crop Science and Resource Conservation (INRES), University of Bonn Rascher et. al. (2015) Sun-induced fluorescence – a new probe of photosynthesis: First maps from the imaging spectrometer HyPlant Global Change Biology, 21, 4673-4684 https://doi.org/10.1111/gcb.13017 Siegmann et. al. (2019) The High-Performance Airborne Imaging Spectrometer HyPlant—From Raw Images to Top-of-Canopy Reflectance and Fluorescence Products: Introduction of an Automatized Processing Chain Remote Sensing, 11, article no. 2760 https://doi.org/10.3390/rs11232760
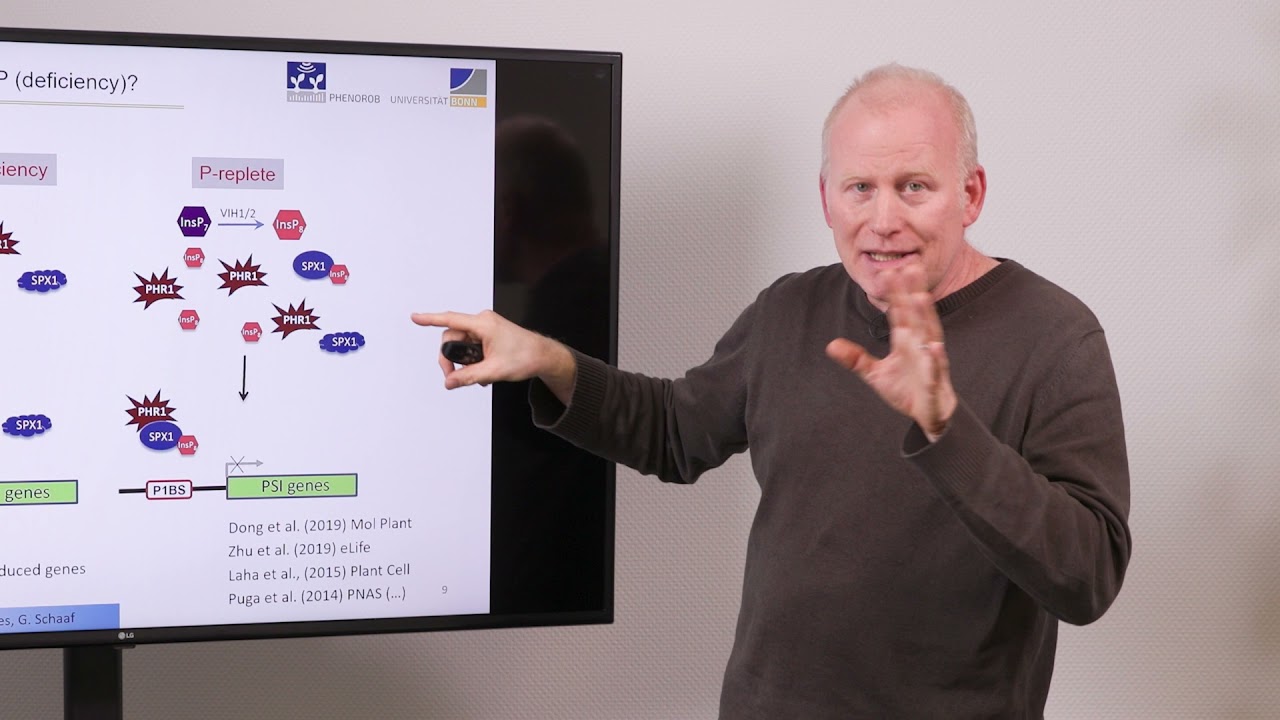
G. Schaaf: ITPK1 is an InsP6/ADP phosphotransferase that controls phosphate signaling in Arabidopsis
Prof. Dr. Gabriel Schaaf is Principal Investigator at PhenoRob and Professor and head of the Ecophysiology of Plant Nutrition Group, Institute of Crop Science and Resource Conservation (INRES), University of Bonn. ITPK1 is an InsP6/ADP phosphotransferase that controls phosphate signaling in Arabidopsis EstherRiemer, Danye Qiu, Debabrata Laha, Robert K.Harmel, Philipp Gaugler, Verena Gaugler, Michael Frei, Mohammad-Reza Hajirezaei, Nargis Parvin Laha, Lukas Krusenbaum, Robin Schneider, Adolfo Saiardi, Dorothea Fiedler, Henning J. Jessen, Gabriel Schaaf, Ricardo F. H. Giehl Molecular Plant Volume 14, Issue 11, 1 November 2021, Pages 1864-1880 https://doi.org/10.1016/j.molp.2021.07.011
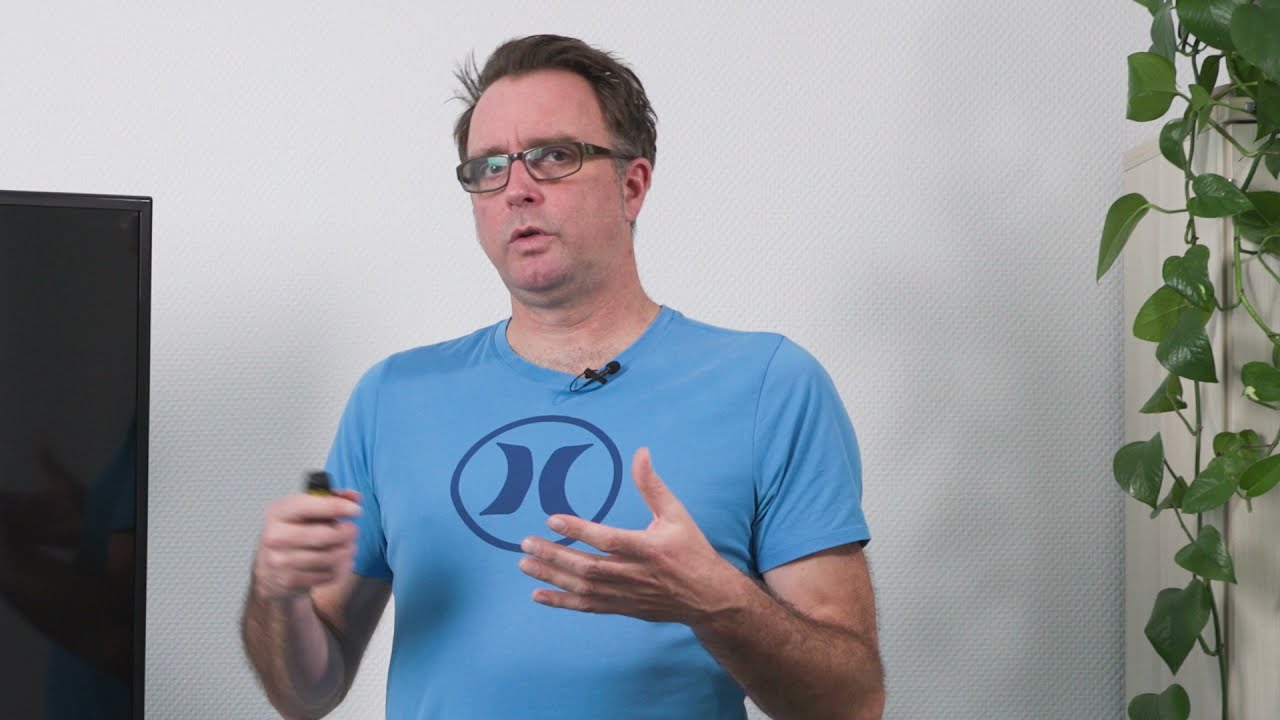
Lasse Klingbeil: Pheno4D: A spatio-temporal dataset of maize and tomato plant point clouds…
Dr. Lasse Klingbeil is Postdoc at the Institute of Geodesy and Geoinformation (IGG), University of Bonn and PhenoRob Member. Pheno4D: A spatio-temporal dataset of maize and tomato plant point clouds for phenotyping and advanced plant analysis D. Schunck, F. Magistri, R. A. Rosu, A. Cornelißen, N. Chebrolu, S. Paulus, J. Léon, S. Behnke, C. Stachniss, H. Kuhlmann, and L. Klingbeil PLOS ONE, vol. 16, iss. 8, pp. 1-18, 2021 Paper: https://doi.org/10.1371/journal.pone.0256340 Data: https://www.ipb.uni-bonn.de/data/pheno4d/
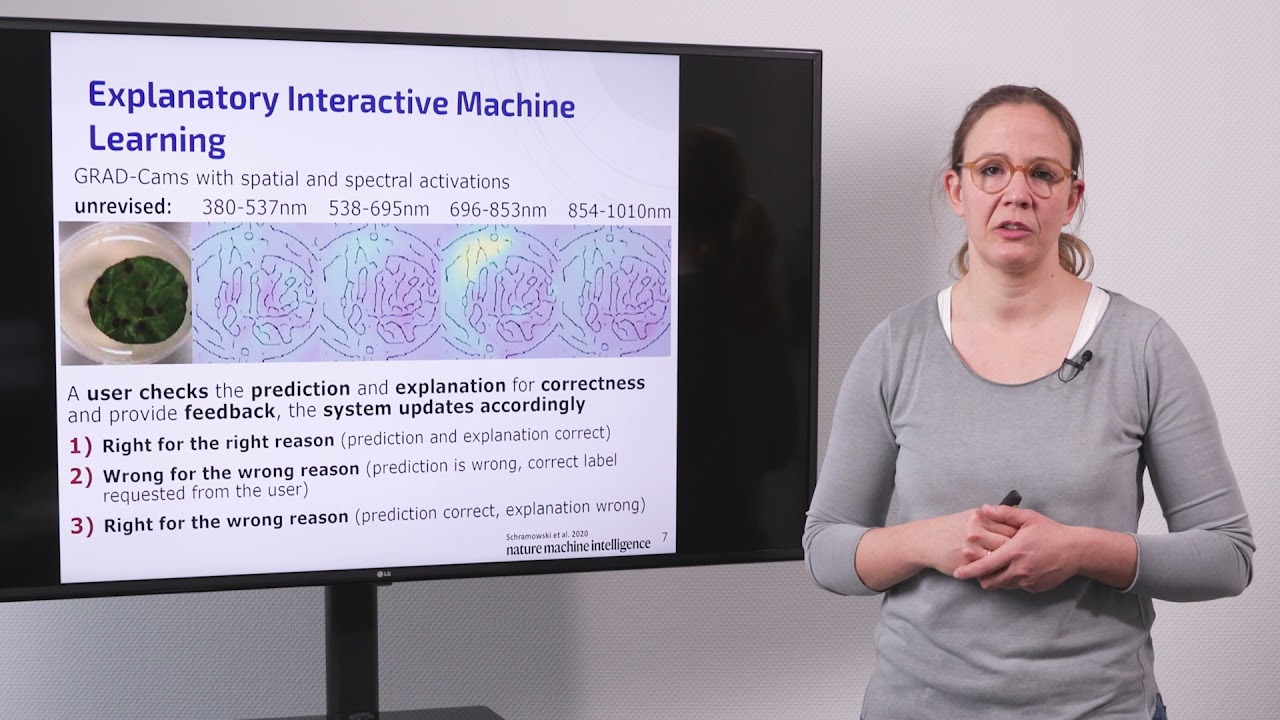
A.-K. Mahlein: Making Deep Neural Networks Right for the Right Scientific Reason…
Prof. Dr. Anne-Katrin Mahlein is Principal Investigator at PhenoRob and Director of the Institute of Sugarbeet Research (IfZ) at the University of Göttingen. Making deep neural networks right for the right scientific reasons by interacting with their explanations. Schramowski, P., Stammer, W., Teso, S. et al. Nat Mach Intell 2, 476–486 (2020). https://doi.org/10.1038/s42256-020-0212-3

Virtual Temporal Samples for RNNs: applied to semantic segmentation in agriculture
Normally, to train a recurrent neural network (RNN), labeled samples from a video (temporal) sequence are required which is laborious and has stymied work in this direction. By generating virtual temporal samples, we demonstrate that it is possible to train a lightweight RNN to perform semantic segmentation on two challenging agricultural datasets. full text in arxiv: https://arxiv.org/abs/2106.10118 check My GitHub for interesting ROS-based projects: https://github.com/alirezaahmadi
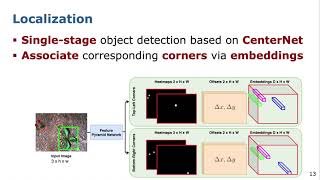
Talk by J. Weyler: Joint Plant Instance Detection and Leaf Count Estimation … (RAL+ICRA’21)
J. Weyler, A. Milioto, T. Falck, J. Behley, and C. Stachniss, “Joint Plant Instance Detection and Leaf Count Estimation for In-Field Plant Phenotyping,” IEEE Robotics and Automation Letters (RA-L), vol. 6, pp. 3599-3606, 2021. doi:10.1109/LRA.2021.3060712 #UniBonn #StachnissLab #robotics #PhenoRob #neuralnetworks #talk
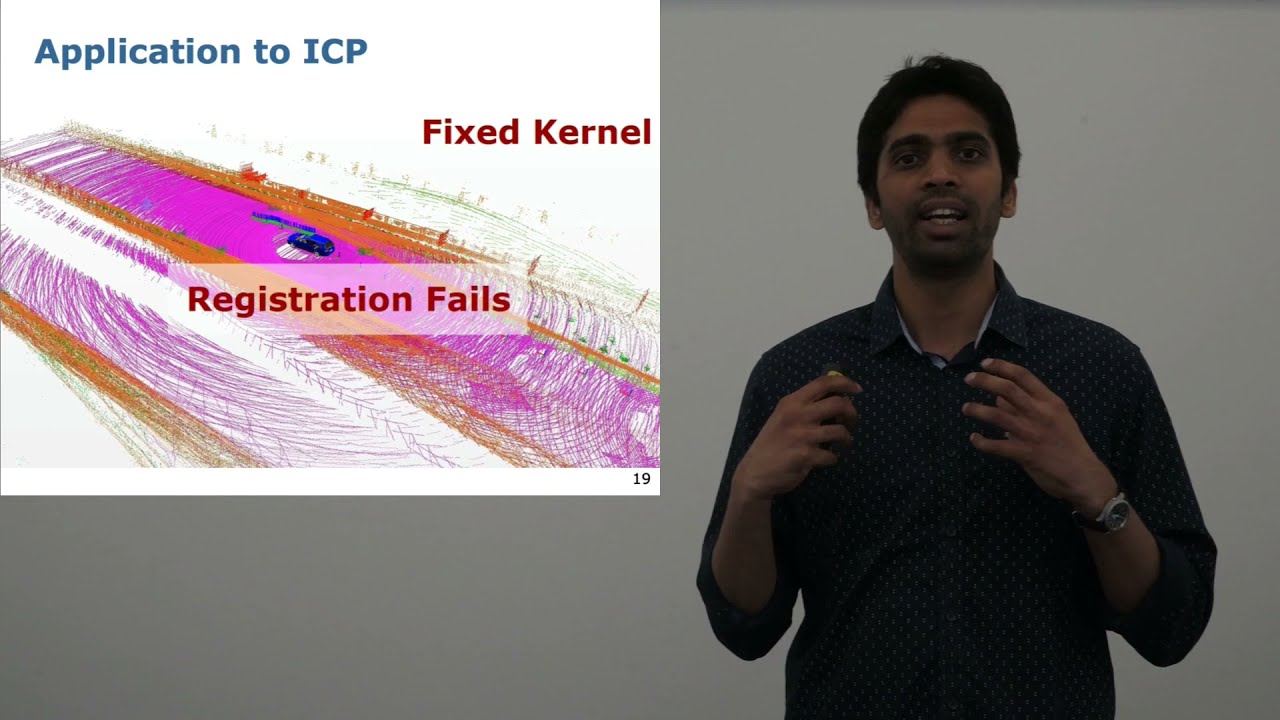
Talk by N. Chebrolu: Adaptive Robust Kernels for Non-Linear Least Squares Problems (RAL+ICRA’21)
N. Chebrolu, T. Läbe, O. Vysotska, J. Behley, and C. Stachniss, “Adaptive Robust Kernels for Non-Linear Least Squares Problems,” IEEE Robotics and Automation Letters (RA-L), vol. 6, pp. 2240-2247, 2021. doi:10.1109/LRA.2021.3061331 https://www.ipb.uni-bonn.de/wp-content/papercite-data/pdf/chebrolu2021ral.pdf #UniBonn #StachnissLab #robotics #autonomouscars #slam #talk
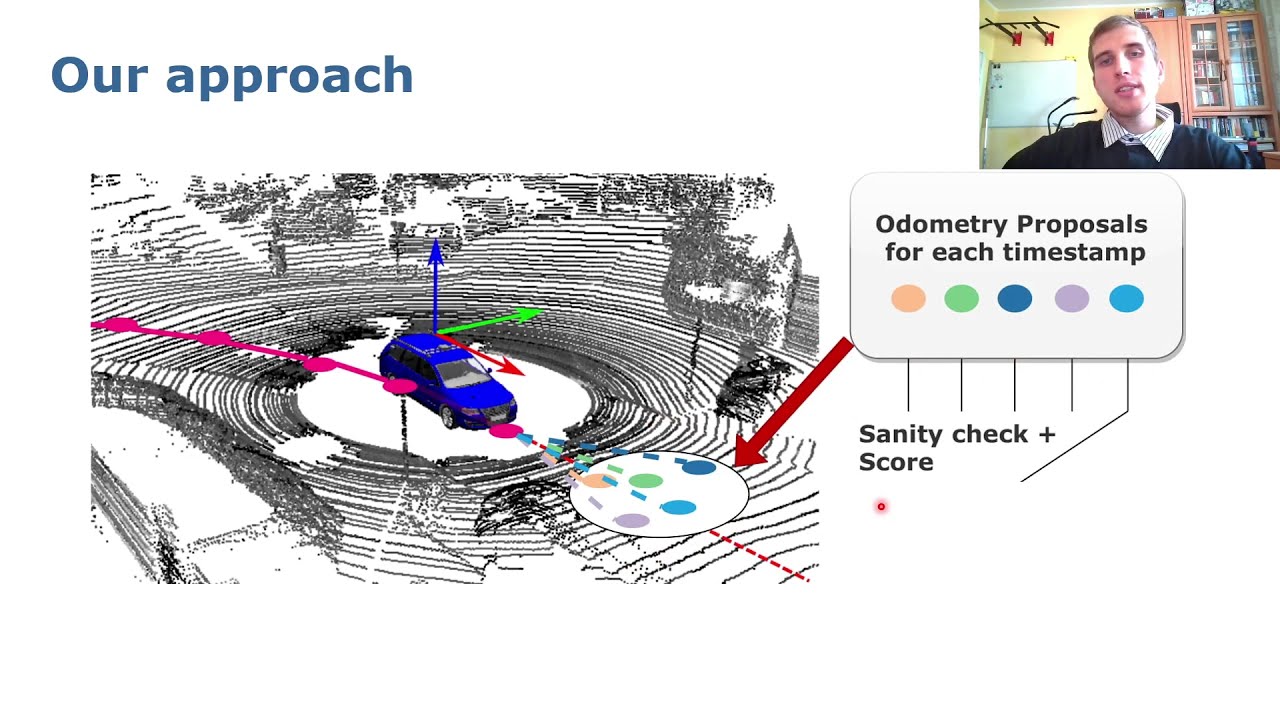
Talk by A. Reinke: Simple But Effective Redundant Odometry for Autonomous Vehicles (ICRA’21)
A. Reinke, X. Chen, and C. Stachniss, “Simple But Effective Redundant Odometry for Autonomous Vehicles,” in Proceedings of the IEEE Int. Conf. on Robotics & Automation (ICRA), 2021. PDF: https://www.ipb.uni-bonn.de/wp-content/papercite-data/pdf/reinke2021icra.pdf Code: https://github.com/PRBonn/MutiverseOdometry #UniBonn #StachnissLab #robotics #autonomouscars #talk
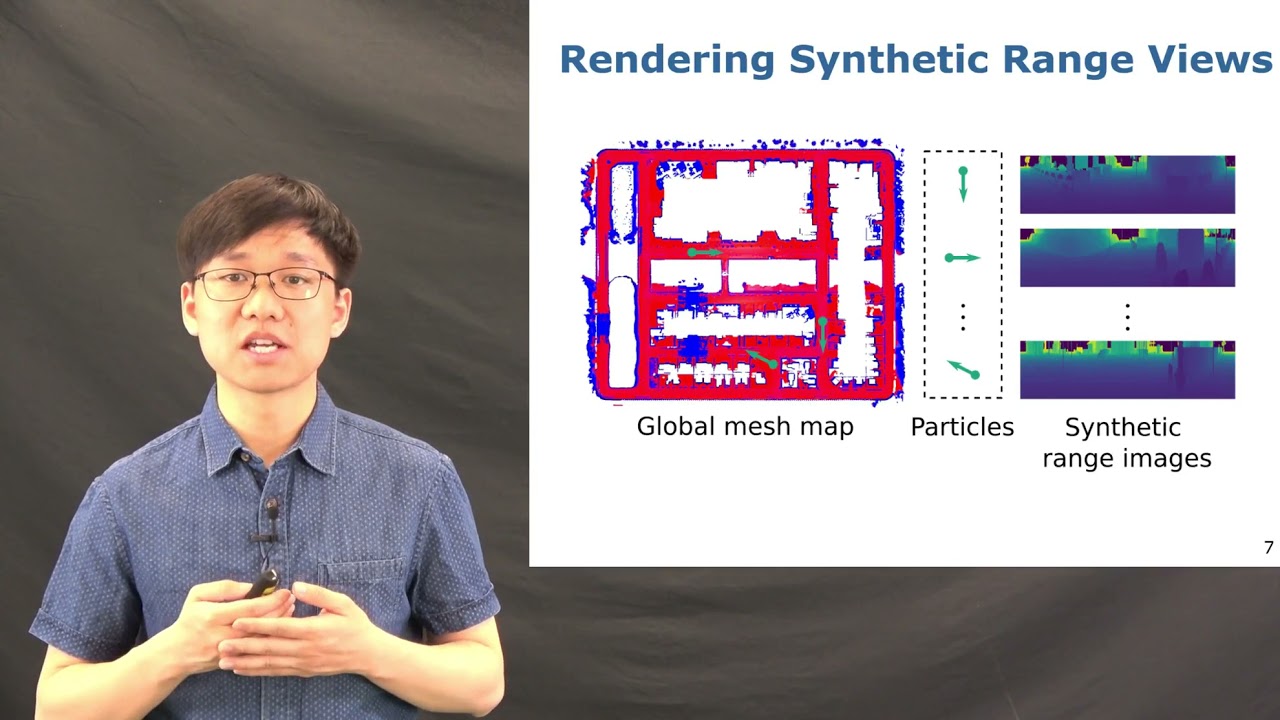
Talk by X. Chen: Range Image-based LiDAR Localization for Autonomous Vehicles (ICRA’21)
X. Chen, I. Vizzo, T. Läbe, J. Behley, and C. Stachniss, “Range Image-based LiDAR Localization for Autonomous Vehicles,” in Proceedings of the IEEE Int. Conf. on Robotics & Automation (ICRA), 2021. Paper: https://www.ipb.uni-bonn.de/wp-content/papercite-data/pdf/chen2021icra.pdf Code: https://github.com/PRBonn/range-mcl #UniBonn #StachnissLab #robotics #autonomouscars #neuralnetworks #talk
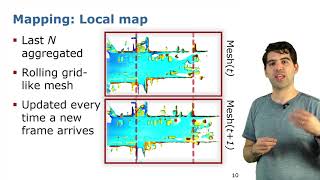
Talk by I. Vizzo: Poisson Surface Reconstruction for LiDAR Odometry and Mapping (ICRA’21)
I. Vizzo, X. Chen, N. Chebrolu, J. Behley, and C. Stachniss, “Poisson Surface Reconstruction for LiDAR Odometry and Mapping,” in Proceedings of the IEEE Int. Conf. on Robotics & Automation (ICRA), 2021. Paper: https://www.ipb.uni-bonn.de/wp-content/papercite-data/pdf/vizzo2021icra.pdf Code: https://github.com/PRBonn/puma #UniBonn #StachnissLab #robotics #autonomouscars #slam #talk
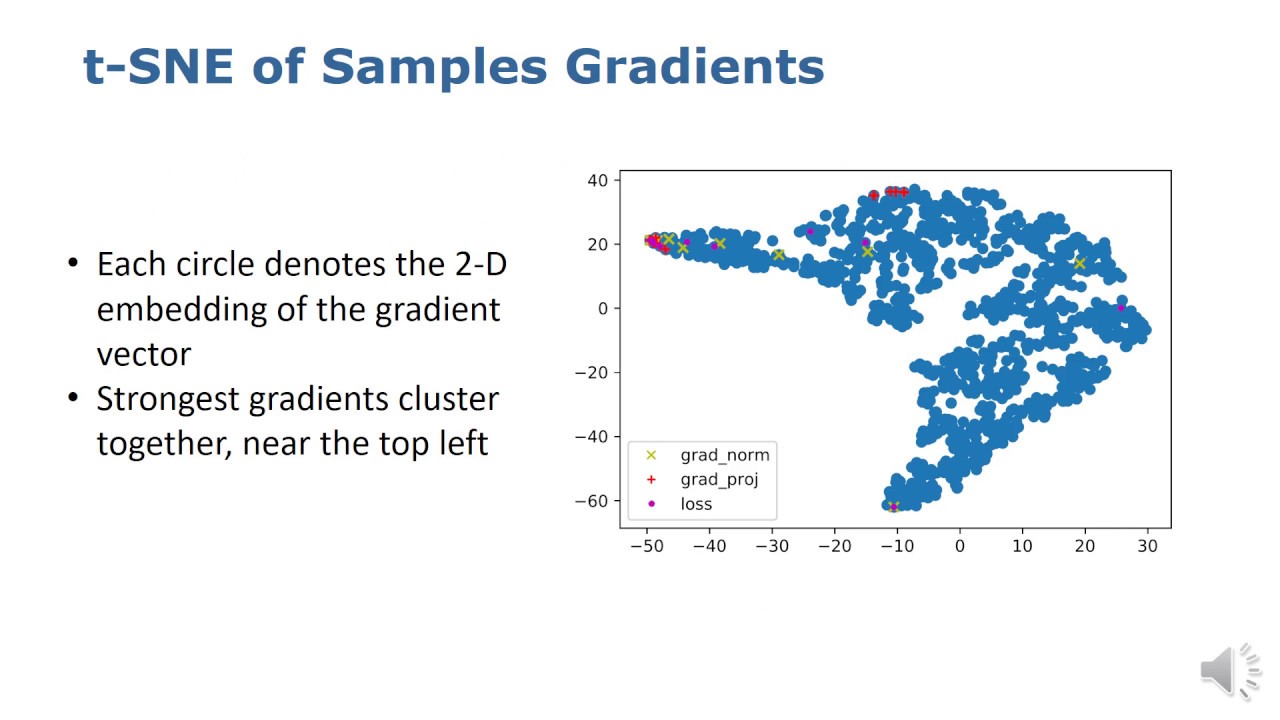
Talk by R. Sheikh on Gradient and Log-based Active Learning for Semantic Segmentation… (ICRA’20)
ICRA 2020 talk about the paper: R. Sheikh, A. Milioto, P. Lottes, C. Stachniss, M. Bennewitz, and T. Schultz, “Gradient and Log-based Active Learning for Semantic Segmentation of Crop and Weed for Agricultural Robots,” in Proceedings of the IEEE Int. Conf. on Robotics & Automation (ICRA), 2020. PDF: https://www.ipb.uni-bonn.de/wp-content/papercite-data/pdf/sheikh2020icra.pdf
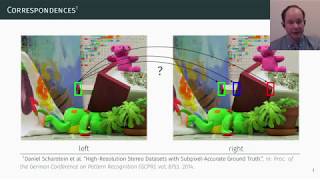
Talk by J. Quenzel on Beyond Photometric Consistency: Gradient-based Dissimilarity for VO (ICRA’20)
ICRA 2020 talk about the paper: J. Quenzel, R. A. Rosu, T. Laebe, C. Stachniss, and S. Behnke, “Beyond Photometric Consistency: Gradient-based Dissimilarity for Improving Visual Odometry and Stereo Matching,” in Proceedings of the IEEE Int. Conf. on Robotics & Automation (ICRA), 2020. PDF: http://www.ipb.uni-bonn.de/pdfs/quenzel2020icra.pdf
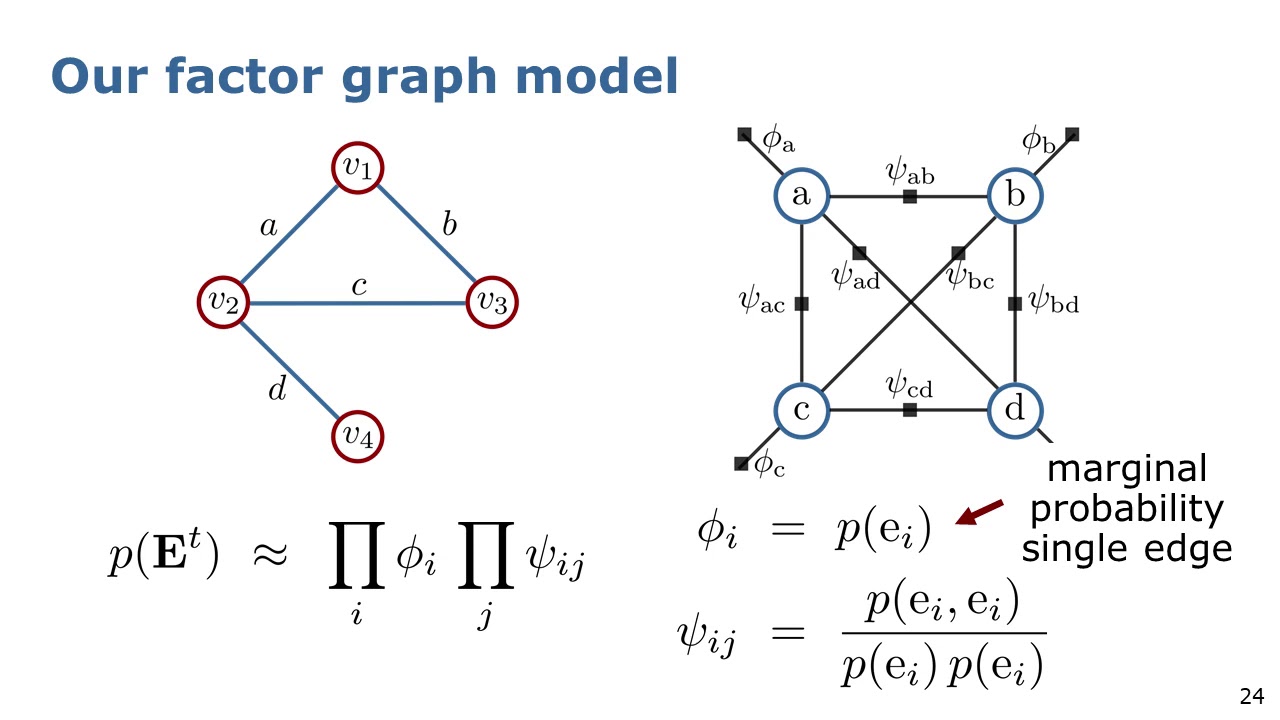
Talk by L. Nardi on Long-Term Robot Navigation in Indoor Environments… (ICRA’20)
ICRA 2020 talk about the paper: L. Nardi and C. Stachniss, “Long-Term Robot Navigation in Indoor Environments Estimating Patterns in Traversability Changes,” in Proceedings of the IEEE Int. Conf. on Robotics & Automation (ICRA), 2020. PDF: https://www.ipb.uni-bonn.de/wp-content/papercite-data/pdf/nardi2020icra.pdf Discussion Slack Channel: https://icra20.slack.com/app_redirect?channel=moa08_1
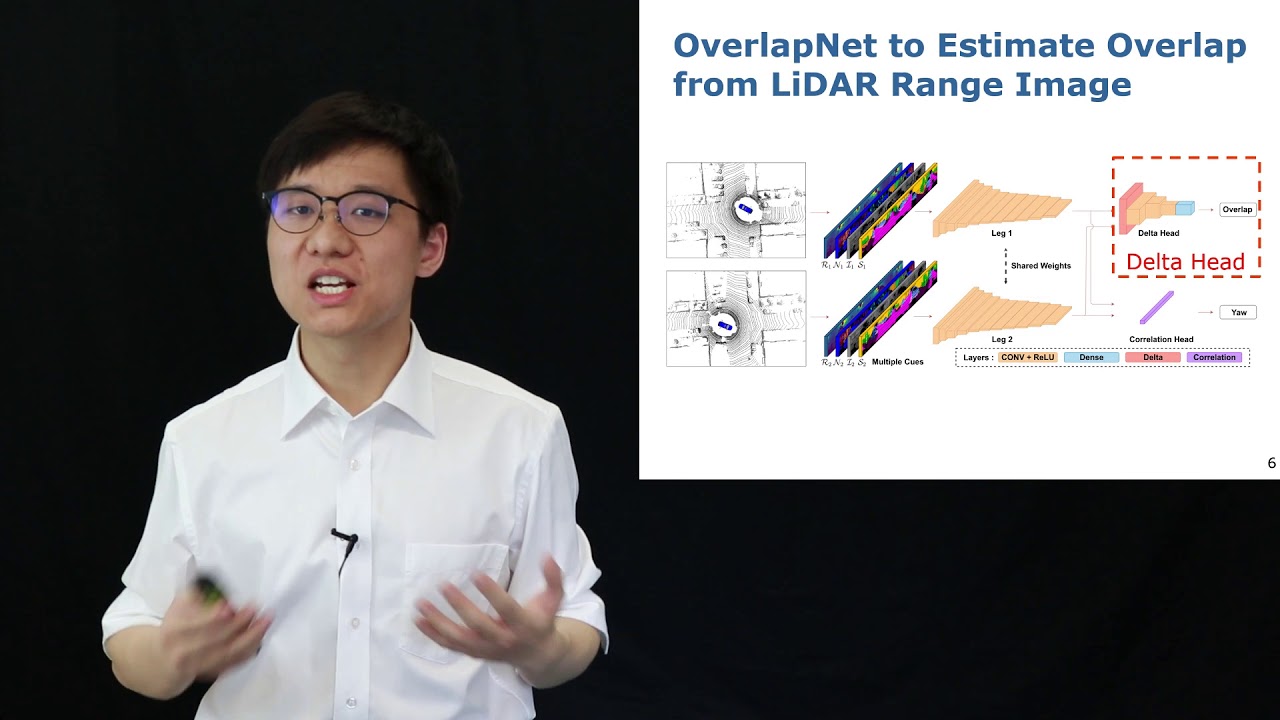
Talk by X. Chen on OverlapNet – Loop Closing for LiDAR-based SLAM (RSS’20)
Talk for the RSS 2020 paper: X. Chen, T. Läbe, A. Milioto, T. Röhling, O. Vysotska, A. Haag, J. Behley, and C. Stachniss, “OverlapNet: Loop Closing for LiDAR-based SLAM,” in Proceedings of Robotics: Science and Systems (RSS), 2020. Paper: https://www.ipb.uni-bonn.de/wp-content/papercite-data/pdf/chen2020rss.pdf Code available: https://github.com/PRBonn/OverlapNet

IROS’20: Segmentation-Based 4D Registration of Plants Point Clouds for Phenotyping by Magistri et al
F. Magistri, N. Chebrolu, and C. Stachniss, “Segmentation-Based 4D Registration of Plants Point Clouds ,” in Proceedings of the IEEE/RSJ Int. Conf. on Intelligent Robots and Systems (IROS), 2020. Paper: https://www.ipb.uni-bonn.de/wp-content/papercite-data/pdf/magistri2020iros.pdf #UniBonn #StachnissLab #robotics #PhenoRob #talk

Talk by D. Gogoll: Unsupervised Domain Adaptation for Transferring Plant Classification…(IROS’20)
D. Gogoll, P. Lottes, J. Weyler, N. Petrinic, and C. Stachniss, “Unsupervised Domain Adaptation for Transferring Plant Classification Systems to New Field Environments, Crops, and Robots,” in Proceedings of the IEEE/RSJ Int. Conf. on Intelligent Robots and Systems (IROS), 2020. PAPER: https://www.ipb.uni-bonn.de/wp-content/papercite-data/pdf/gogoll2020iros.pdf

IROS’20: LiDAR Panoptic Segmentation for Autonomous Driving presented by J. Behley
Trailer video for the paper: A. Milioto, J. Behley, C. McCool, and C. Stachniss, “LiDAR Panoptic Segmentation for Autonomous Driving,” in Proceedings of the IEEE/RSJ Int. Conf. on Intelligent Robots and Systems (IROS), 2020. Paper: https://www.ipb.uni-bonn.de/wp-content/papercite-data/pdf/milioto2020iros.pdf
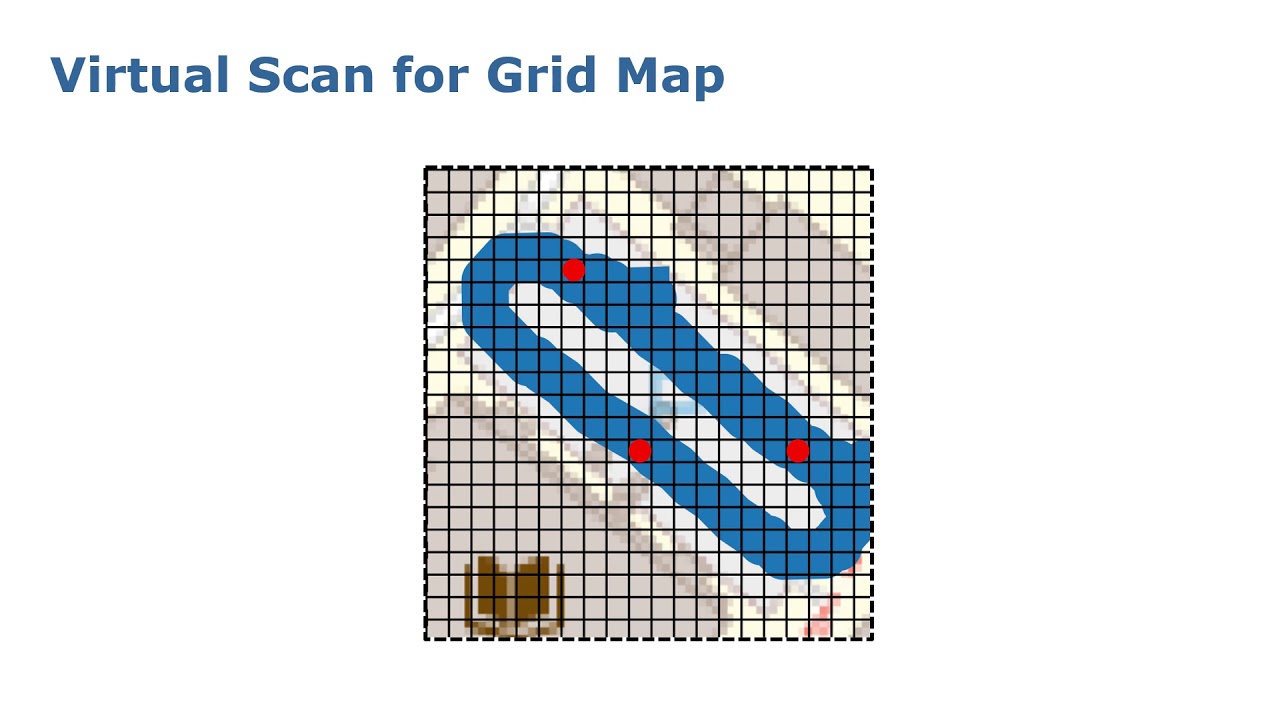
IROS’20: Learning an Overlap-based Observation Model for 3D LiDAR Localization by Chen et al.
Trailer Video for the work: X. Chen, T. Läbe, L. Nardi, J. Behley, and C. Stachniss, “Learning an Overlap-based Observation Model for 3D LiDAR Localization,” in Proceedings of the IEEE/RSJ Int. Conf. on Intelligent Robots and Systems (IROS), 2020. Paper: https://www.ipb.uni-bonn.de/wp-content/papercite-data/pdf/chen2020iros.pdf Code available!
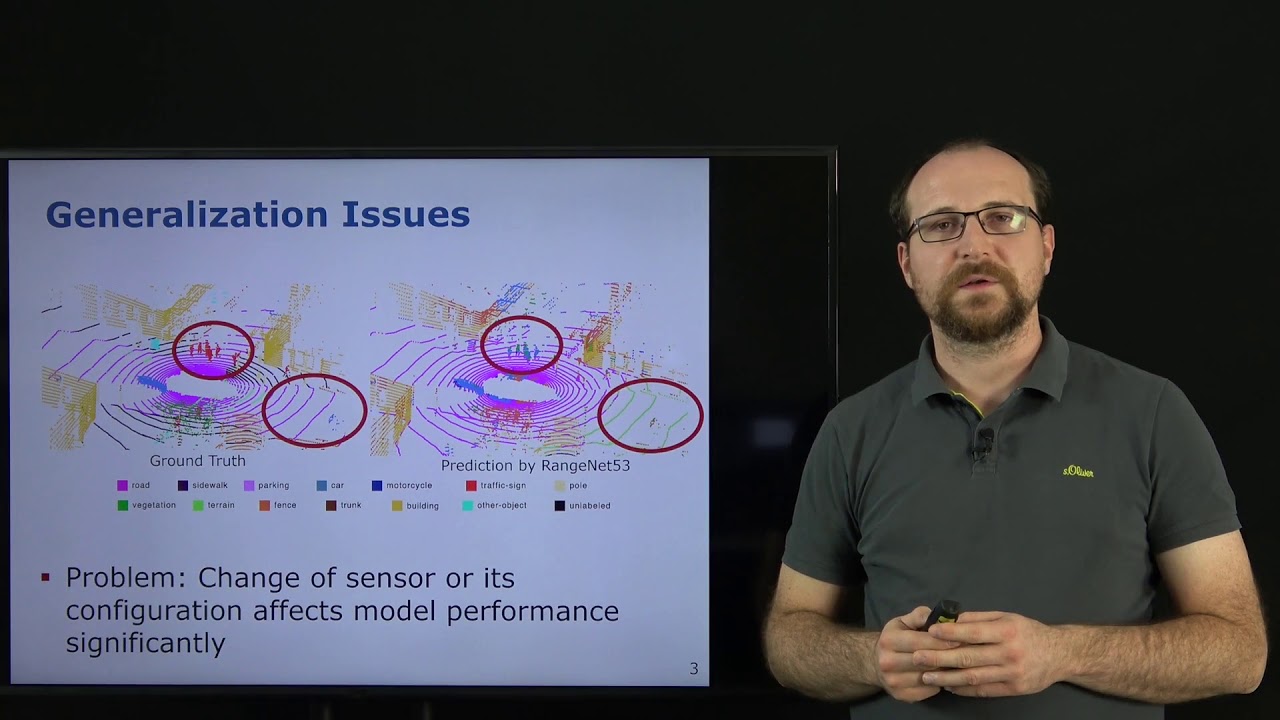
IROS’20: Domain Transfer for Semantic Segmentation of LiDAR Data using DNNs presented by J. Behley
F. Langer, A. Milioto, A. Haag, J. Behley, and C. Stachniss, “Domain Transfer for Semantic Segmentation of LiDAR Data using Deep Neural Networks,” in Proceedings of the IEEE/RSJ Int. Conf. on Intelligent Robots and Systems (IROS), 2020. Paper: https://www.ipb.uni-bonn.de/wp-content/papercite-data/pdf/langer2020iros.pdf
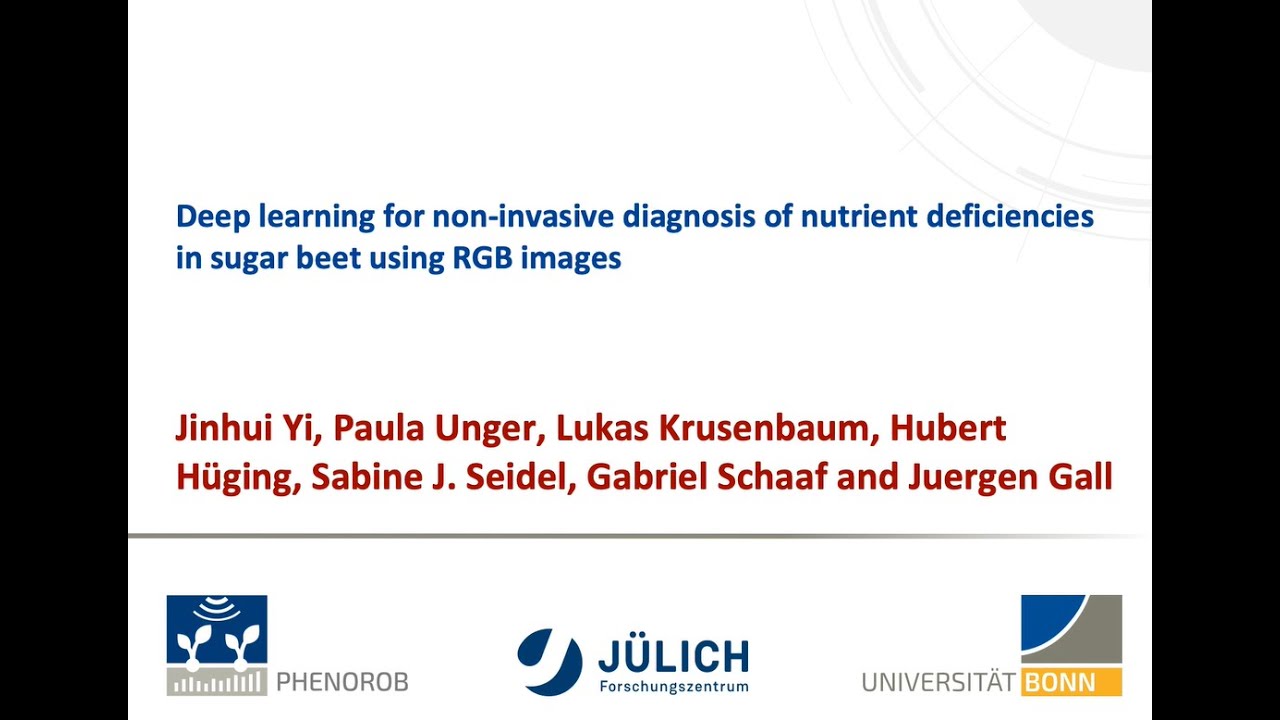
Deep Learning for Non-Invasive Diagnosis of Nutrient Deficiencies in Sugar Beet Using RGB Images
Yi, J.; Krusenbaum, L.; Unger, P.; Hüging, H.; Seidel, S.J.; Schaaf, G.; Gall, J. Deep Learning for Non-Invasive Diagnosis of Nutrient Deficiencies in Sugar Beet Using RGB Images. Sensors 2020, 20, 5893. https://www.mdpi.com/1424-8220/20/20/5893
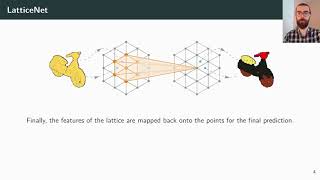
RSS 2020′: LatticeNet: Fast Point Cloud Segmentation Using Permutohedral Lattices
LatticeNet: Fast Point Cloud Segmentation Using Permutohedral Lattices by Radu Alexandru Rosu, Peer Schütt, Jan Quenzel, Sven Behnke Deep convolutional neural networks (CNNs) have shown outstanding performance in the task of semantically segmenting images. However, applying the same methods on 3D data still poses challenges due to the heavy memory requirements and the lack of structured data. Here, we propose LatticeNet, a novel approach for 3D semantic segmentation, which takes as input raw point clouds. A PointNet describes the local geometry which we embed into a sparse permutohedral lattice. The lattice allows for fast convolutions while keeping a low memory footprint. Further, we introduce DeformSlice, a novel learned data-dependent interpolation for projecting lattice features back onto the point cloud. We present results of 3D segmentation on various datasets where our method achieves state-of-the-art performance.
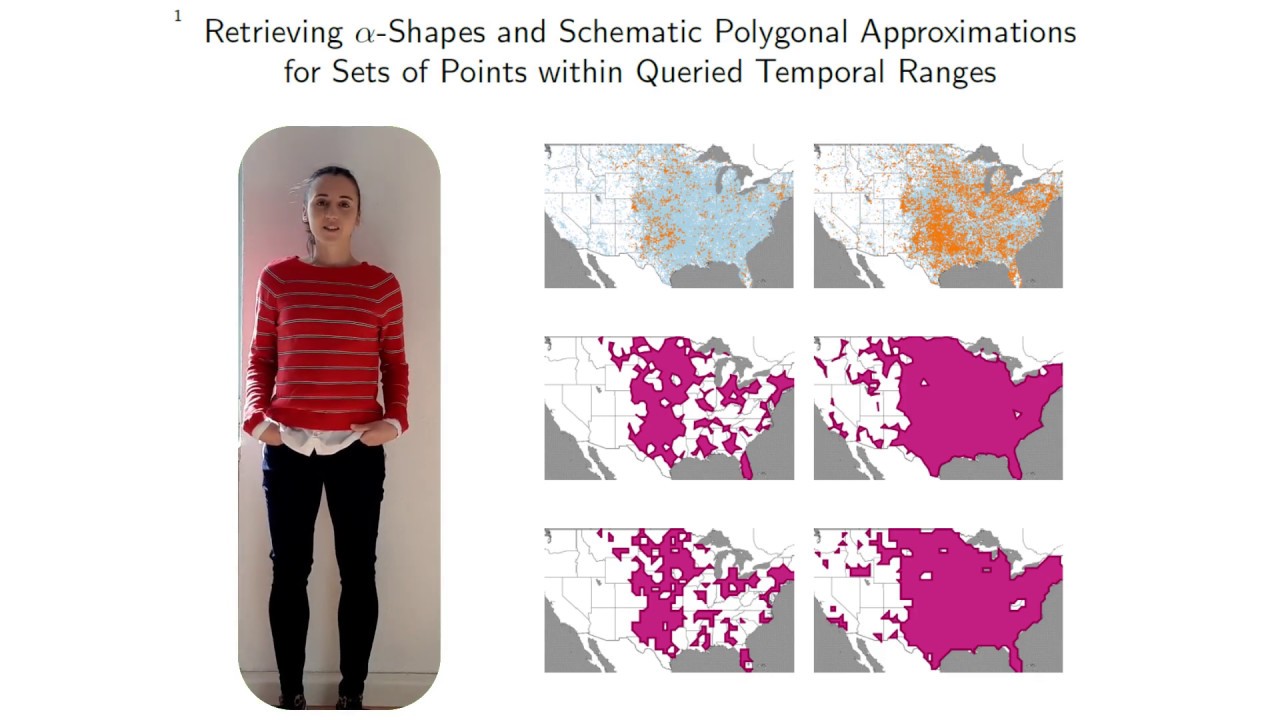
SIGSPATIAL’2019: Retrieving alpha-Shapes and Schematic Polygonal Approximations for Sets of Points..
Retrieving alpha-Shapes and Schematic Polygonal Approximations for Sets of Points within Queried Temporal Ranges by A. Bonerath, B. Niedermann und J.-H. Haunert In Proceedings of the 27th ACM SIGSPATIAL International Conference on Advances in Geographic Information Systems, 2019

ICRA’2020: Visual Servoing-based Navigation for Monitoring Row-Crop Fields
Visual Servoing-based Navigation for Monitoring Row-Crop Fields by A. Ahmadi et al. In Proc. of the IEEE Intl. Conf. on Robotics & Automation (ICRA) , 2020.
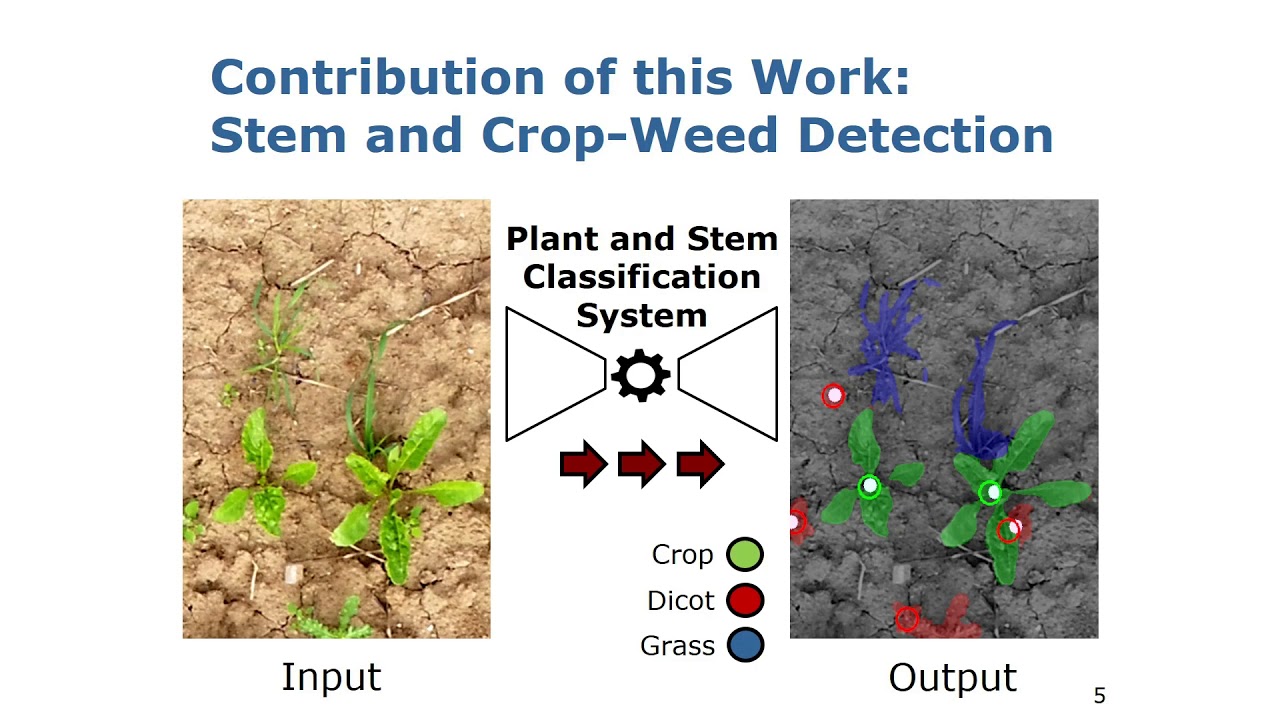
IROS’18: Joint Stem Detection and Crop-Weed Classification for Plant-specific Treatment
Trailer for the paper: Joint Stem Detection and Crop-Weed Classification for Plant-specific Treatment in Precision Farming by P. Lottes, J. Behley, N. Chebrolu, A. Milioto, and C. Stachniss, IROS 2018.
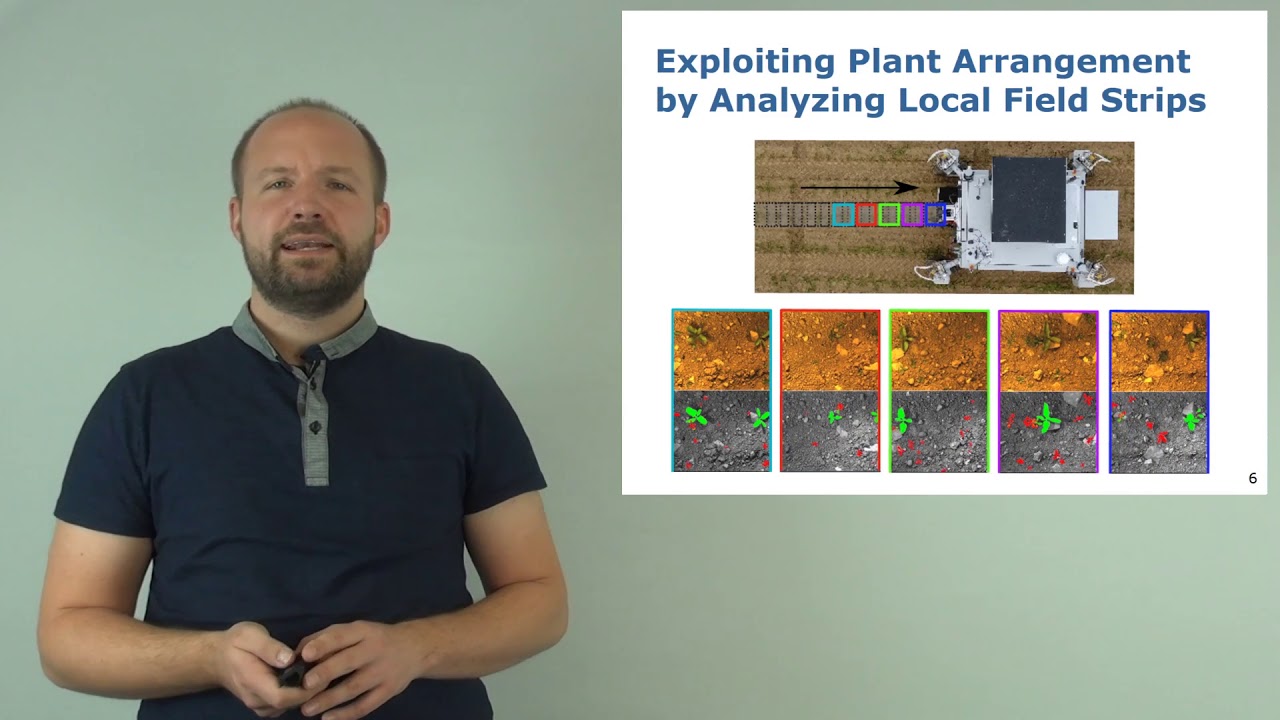
RAL’18: FCNs with Sequential Information for Robust Crop and Weed Detection by Lottes et al.
Trailer for the paper: Fully Convolutional Networks with Sequential Information for Robust Crop and Weed Detection in Precision Farming by P. Lottes, J. Behley, A. Milioto, and C. Stachniss, RAL 2018
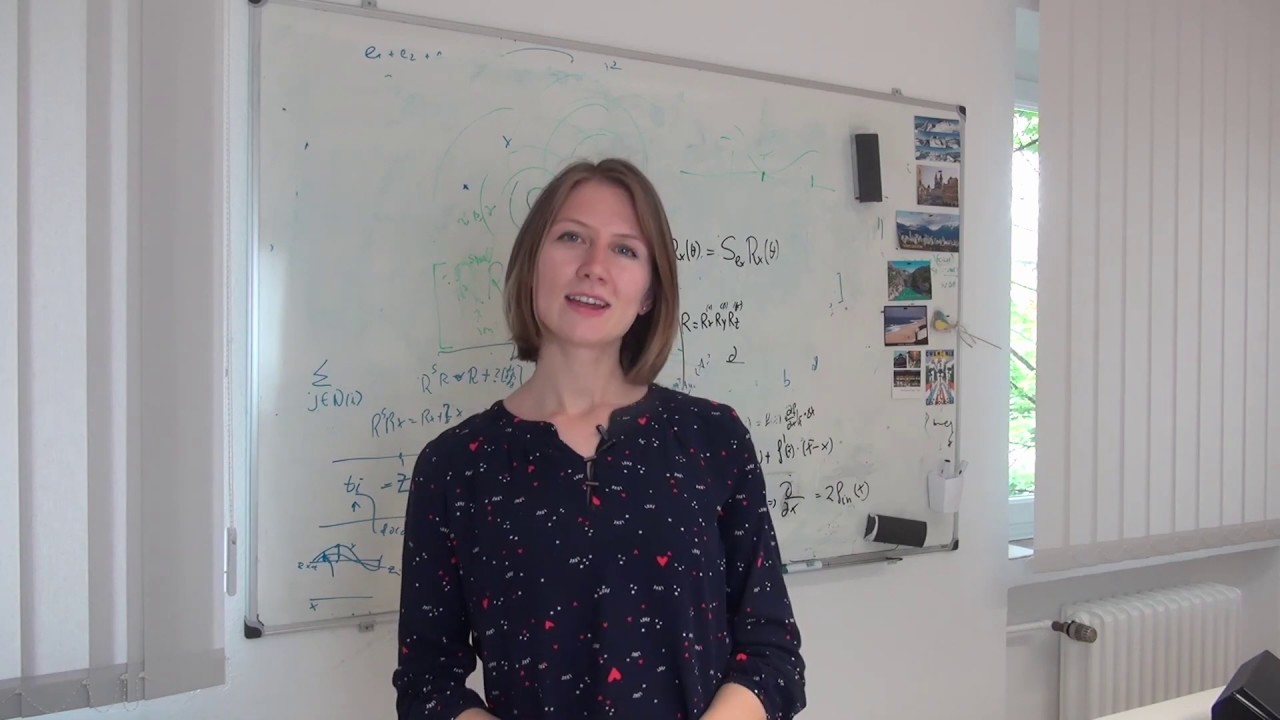
RAL-ICRA’19: Effective Visual Place Recognition Using Multi-Sequence Maps by Vysotska & Stachniss
O. Vysotska and C. Stachniss, “Effective Visual Place Recognition Using Multi-Sequence Maps,” IEEE Robotics and Automation Letters (RA-L) and presentation at ICRA, 2019. PDF: http://www.ipb.uni-bonn.de/wp-content/papercite-data/pdf/vysotska2019ral.pdf

ICRA’19: Actively Improving Robot Navigation On Different Terrains Using GPMMs by Nardi et al.
L. Nardi and C. Stachniss, “Actively Improving Robot Navigation On Different Terrains Using Gaussian Process Mixture Models,” in Proc. of the IEEE Intl. Conf. on Robotics & Automation (ICRA) , 2019. http://www.ipb.uni-bonn.de/wp-content/papercite-data/pdf/nardi2019icra-airn.pdf
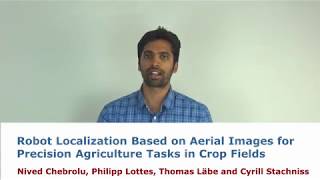
ICRA’19: Robot Localization Based on Aerial Images for Precision Agriculture by Chebrolu et al.
Robot Localization Based on Aerial Images for Precision Agriculture Tasks in Crop Fields by N. Chebrolu, P. Lottes, T. Laebe, and C. Stachniss In Proc. of the IEEE Intl. Conf. on Robotics & Automation (ICRA) , 2019. Paper: http://www.ipb.uni-bonn.de/wp-content/papercite-data/pdf/chebrolu2019icra.pdf